Mastering A/B Testing for Business Success
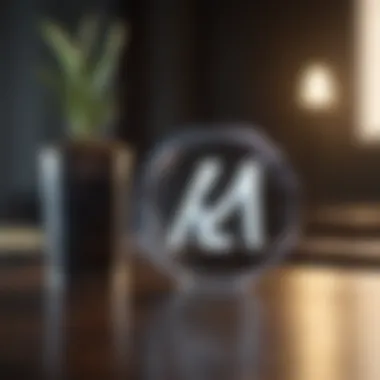
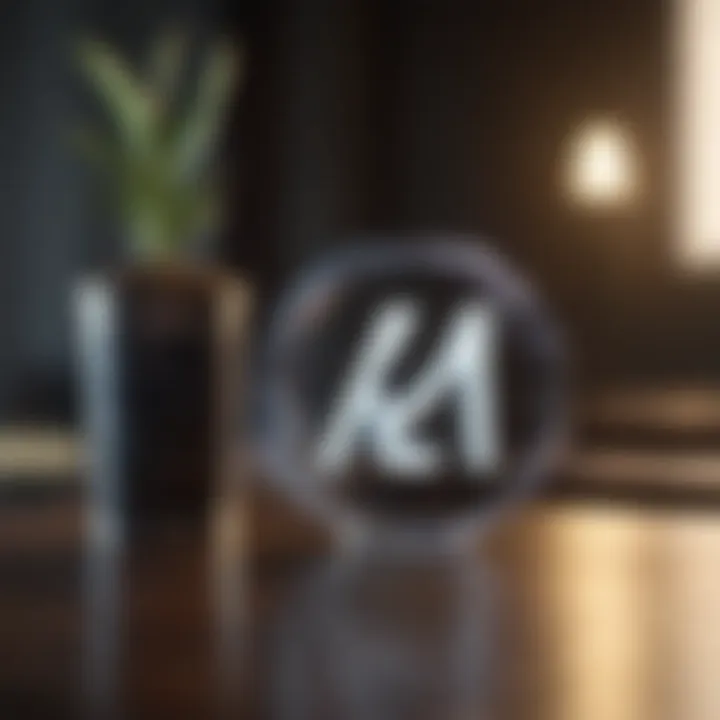
Intro
A/B testing, often regarded as the backbone of data-driven decision-making, has gained significant traction in today's digital landscape. Within various sectors, from e-commerce to software development, businesses increasingly rely on this methodology to refine their strategies and improve user engagement. At its core, A/B testing consists of comparing two versions of a webpage, advertisement, email, or any other asset to discern which one performs better based on specific metrics. This fundamental technique provides valuable insights that can steer any organization’s future actions, making comprehensible the necessity of mastering its principles.
In this article, we’ll embark on a journey through A/B testing, inviting both novice and seasoned marketers to grasp its finer nuances. We’ll cover the basic concepts, highlight the significance it holds in modern marketing tactics, delve into common pitfalls to sidestep, and illustrate practical applications that can elevate your strategy.
Additionally, we will distinguish A/B testing from multivariate testing—a similar, yet distinctly different approach—while sharing best practices to ensure success in your testing campaigns. The role of statistical analysis in confirming credible results cannot be overstated, and we shall elucidate this critical aspect as well.
As we navigate through this invaluable toolkit for making informed decisions, expect to emerge armed with knowledge that holds the potential to positively impact your work outcomes.
A/B Testing Fundamentals
Key Points to Consider
- What Exactly is A/B Testing? A/B testing is often defined as a controlled experiment that compares two versions of a single variable.
- Why Conduct A/B Testing? By analyzing results from A/B tests, one can make data-driven decisions that enhance user experience and drive marketing success.
- The Process: Typically involves the creation of two variations, known as A (the control) and B (the variant), while ensuring that only one element is altered between them, enabling a fair comparison.
Importance in Marketing Strategy
In today's fast-paced digital environment, the significance of A/B testing lies in its capability to cater to the needs of consumers more efficiently. Organizations that apply A/B testing can tailor their marketing initiatives, optimizing channels such as email campaigns, landing pages, and product offers. This process not only improves conversion rates but also assists in minimizing customer acquisition costs.
Distinguishing A/B Testing from Multivariate Testing
The Differences
- Scope of Testing: A/B testing focuses on one variable at a time, while multivariate testing examines multiple variables simultaneously.
- Complexity: Due to its singular focus, A/B testing is generally easier to interpret and manage compared to multivariate testing, which can lead to complex analysis.
"A/B testing is the art of comparing apples and oranges in a world full of fruit salads, allowing you to determine which has a certain zest that your audience craves."
Best Practices for A/B Testing
When embarking on your A/B testing endeavor, consider the following insights to promote success:
- Define Clear Objectives: Start by determining what you want to achieve with your test.
- Segment Your Audience: Analyze different user groups to uncover unique preferences.
- Be Patient: Allow your tests to run long enough to collect sufficient data. )
Role of Statistical Analysis
Statistics might seem daunting at first glance, but its significance becomes clear when interpreting A/B testing results. The essence of statistical analysis is to discern whether observed changes are due to the test or merely incidental fluctuations. By employing methods like confidence levels and p-values, marketers can draw solid conclusions that inform their decisions.
This investment in understanding A/B testing can certainly yield dividends. As we explore the practical applications and the intricacies involved, you'll gain insights that empower strategic choices for your marketing efforts.
Prelims to A/B Testing
In the world of marketing and product development, understanding how different changes affect user behavior can make or break a business. This is where A/B testing shines as a fundamental pillar of data-driven decision-making. It provides a clear, evidence-based way to evaluate alternatives. Instead of relying on instinct or guesswork, A/B testing offers concrete data to support decisions that can enhance user experience or boost conversion rates.
By isolating variables, A/B testing allows companies to test one element at a time. The beauty of it is in simplicity: by showing one group a version 'A' and another group a version 'B', you can directly measure which variation performs better. This clarity is essential for marketers and product developers who are constantly trying to fine-tune their approaches to meet consumer needs.
Key Elements of A/B Testing:
- Data-Driven Decisions: Helps businesses minimize risk by backing their choices with data rather than intuition.
- Measurable Outcomes: Results are quantifiable, allowing companies to track success over time.
- Iterative Improvement: Continuous testing leads to ongoing optimization of products or marketing strategies.
Considerations regarding A/B testing include the importance of defining clear metrics for success and choosing practical variables to test. Knowing what you aim to improve, whether that’s website traffic, email open rates, or customer engagement, shapes the design of the test.
Importantly, A/B testing is not just a one-off exercise. It’s a mindset that encourages regular evaluation and adaptation. Marketers and businesses can leverage the insights gained from each test to refine future strategies, ultimately leading to more informed decisions that can significantly impact their bottom line.
"In marketing, if you’re not testing, you’re guessing. A/B testing takes the guesswork out of success.”
As we transition into the core of A/B testing, it becomes crucial to explore its definition and underlying principles.
The Mechanics of A/B Testing
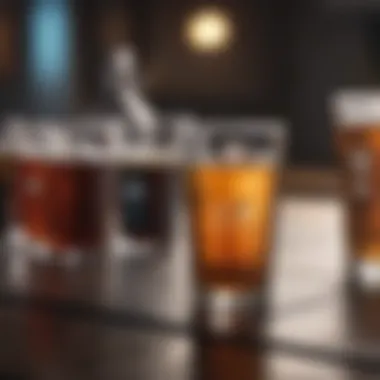
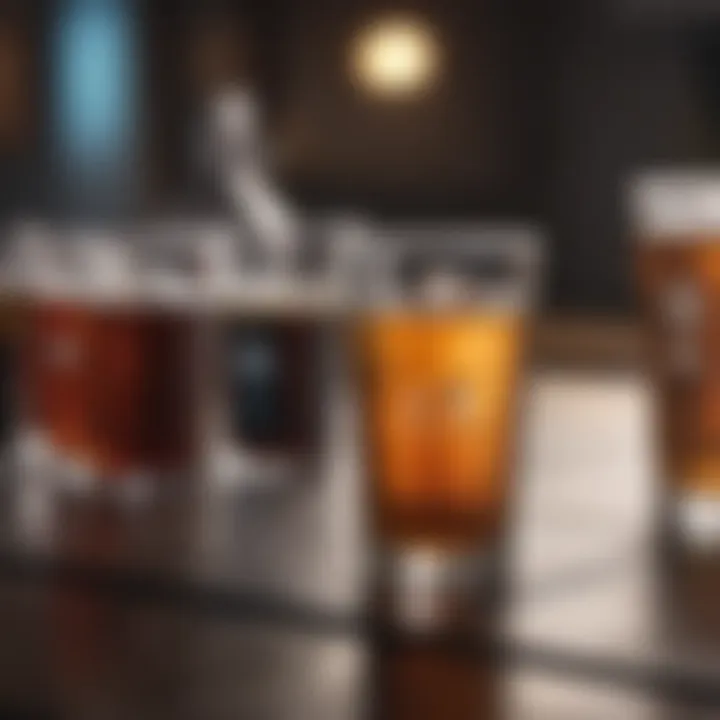
Understanding the mechanics of A/B testing is like peeling an onion; there are layers that all contribute to effective decision-making based on user data. A/B testing allows businesses to fine-tune strategies with precision. By comparing two versions of a webpage or a marketing email, it reveals which one resonates more with the target audience. This systematic approach not only optimizes user engagement but also drives actionable insights into consumer behavior.
How A/B Testing Works
To grasp A/B testing fully, one must understand its nuts and bolts. The process begins with the creation of two variations of a single variable; these can be anything from a headline to a call-to-action button. The audience is then split into two groups: one sees version A while the other sees version B. Metrics such as click-through rates, purchase conversions, and bounce rates are monitored to assess performance.
It’s important to note that A/B testing operates on the principle of statistical analysis. It’s not just about which version looks better; it’s about solid numbers that, when interpreted correctly, guide marketing campaigns more effectively. For a marketer, being data-driven in choices based on these results can be a game-changer.
Setting Up an A/B Test
Choosing a Variable to Test
The heart of any A/B test is the variable. This could be as simple as changing the color of a button or revising the wording in a headline. The significance lies in identifying what needs to be improved. Picking a variable to test is a crucial step that shapes the outcome of the entire experiment. It’s essential to select a variable that is not only relevant but manageable within the context of the campaign.
For instance, suppose a retail website is seeing low conversion rates on a product page. Testing the "Add to Cart" button color could shed light on whether a more vibrant hue will improve user engagement. The key characteristic here is focusing on one element to isolate its direct impact, which is beneficial for drawing clear conclusions.
Defining Success Metrics
Success metrics are the measuring stick for A/B testing, illuminating whether the test is making a tangible impact. This might include metrics like increase in sales, user engagement, or lead generation. Choosing the right metrics can mean the difference between actionable insights and mere statistics you can't leverage.
When launching an A/B test, it’s crucial to articulate how success will be quantified. If an email campaign tests two subjects lines, open rates become the relevant success metric. The unique feature of defining success metrics means clarifying what constitutes winning and losing, which leads to a sharper focus on outcomes.
Determining Sample Size
The next crucial step involves determining how large the sample size should be—this influences the reliability of your results. Too small a sample might yield misleading data while a sample that’s too large could waste resources.
A good practice is to calculate the required sample size based on the expected conversion rate and desired confidence level. This allows for more accurate predictions regarding the impact of changes made. The unique feature here is that a correctly sized sample enhances the credibility of results, making it easier to pinpoint effective strategies.
Running the Test
Duration and Timing Considerations
Waiting for results requires patience, much like planting seeds and ensuring they get water and sunlight enough to grow. The duration of an A/B test can impact the reliability of its conclusions. A test that runs for too little time may not capture variations in user behavior across different days or times. On the other hand, extending it longer than necessary could lead to diminishing returns.
One must consider factors like traffic levels and seasonality in their timing. If conducting a test during a holiday season, user behaviors may differ significantly compared to regular times. The nuance here is in strategically timing the test to align with natural user patterns, optimizing the potential for valid results.
Randomization Techniques
Randomization is the backbone of A/B testing that ensures each participant in the study has an equal chance of being assigned to either version. This technique helps eliminate biases that may sway results. Without it, external factors could unfairly skew what seems like effective changes.
There are different methods for randomization: simple random sampling or stratified random sampling, for example. The unique feature of using randomization lies in its ability to create a level playing field for the variations being tested, which is essential for the integrity of results.
"Testing and iterating is not just a method; it's a mindset that helps businesses thrive in a rapidly changing landscape."
Analyzing A/B Test Results
A/B testing isn't just about running experiments; the real meat of the process lies in analyzing the results. This phase allows marketers, developers, and decision-makers to draw actionable insights from the data collected. It’s vital to understand that the accuracy of conclusions can make or break user experiences and ultimately impact overall business objectives. By analyzing A/B test results effectively, one can identify what changes yield significant benefits or if they just muddled the waters.
Interpreting Data
Statistical Significance
Statistical significance is at the heart of understanding A/B testing results. It essentially answers the question: "Is the difference observed between variations likely due to chance or actual change?" A common threshold used in testing is the 0.05 level which indicates a 95% confidence that the results are not random flukes. The key characteristic here is that it helps in establishing reliability in the findings. This makes statistical significance a widely accepted standard in the industry.
However, the unique feature of statistical significance is that it doesn’t measure the size of the effect or its practical significance. What feels a bit daunting is that even with statistical significance, the effects can still be small. So, while it tells you the result is likely not due to chance, you still need to assess whether the change is meaningful for your objectives.
Confidence Intervals
Confidence intervals take statistical analysis a step further by providing a range wherein the true population parameter probably lies. They give a better flavor of uncertainty than a single point estimate. A common interval is 95%, meaning you can be confident that your data analysis captures the real impact most of the time.
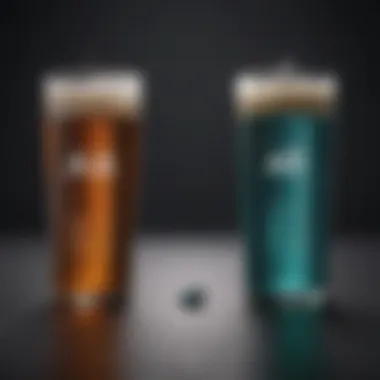
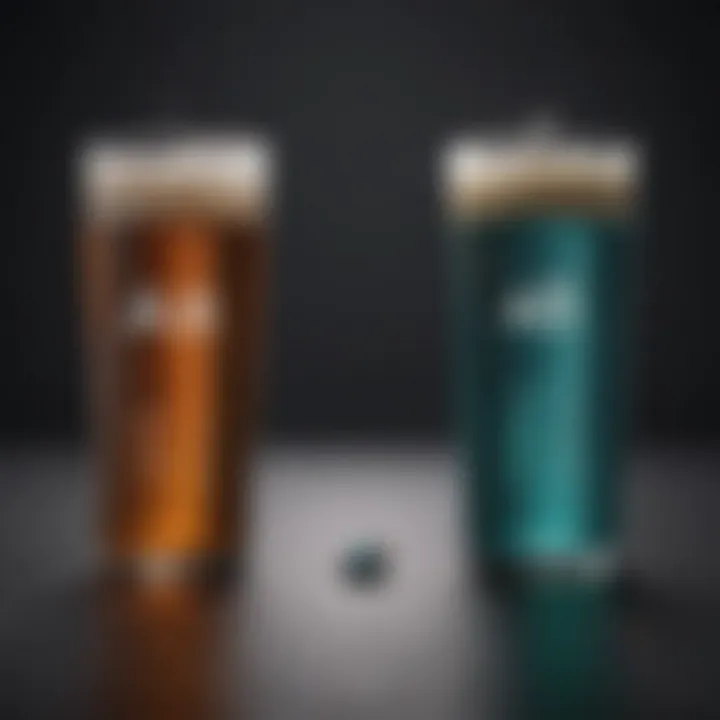
The key characteristic of confidence intervals is that they provide a richer context than just statistical significance alone. Unlike the earlier discussion on statistical significance, confidence intervals allow decision-makers to gauge the precision of the estimates as well. Yet, one downside is they can sometimes be misinterpreted. Amateurs might see a narrow confidence interval as an indicator of a strong result when, in fact, a wider range may also encompass essential data that needs consideration.
Common Statistical Tests Used
In the realm of A/B testing, understanding which statistical tests to employ becomes pivotal. Here are two popular tests:
T-tests
T-tests are commonly employed to compare the means between two groups, thus giving insights into whether the observed differences are statistically meaningful. The key characteristic of T-tests is their ability to handle small sample sizes relatively well, making them a favored option, especially for teams that might not have huge datasets.
A unique feature of T-tests is that they are easy to implement using common statistical software or even Excel. One of the advantages is how T-tests can yield significant insights relatively quickly. However, users should note that they assume a normal distribution, which can lead to misleading results in cases of skewed data distributions. Thus users need to approach with caution and preferably sample sizes that meet this assumption.
Chi-Squared Tests
Chi-squared tests are another go-to choice for A/B testing, especially when dealing with categorical variables. They help assess how the observed counts in categories deviate from the expected counts. The key characteristic here is that it’s particularly effective for larger datasets, where patterns can be clearer and outcomes more reliable.
What’s unique about Chi-squared tests is that they don’t require the data to be normally distributed, which can be a major advantage over T-tests in certain scenarios. However, selecting an appropriate outcome measure is crucial since running a Chi-squared test on small sample sizes can lead to inaccurate conclusions. The challenge is remembering that even with larger datasets, conclusions could still be skewed if the underlying assumptions are not met.
Analyzing the results from A/B tests with statistical significance and an understanding of confidence intervals and tests like T-tests and Chi-squared tests is fundamental to making informed business decisions. Each statistical measure offers unique benefits and drawbacks, making their thoughtful application crucial in the interpretation process.
Best Practices in A/B Testing
When it comes to A/B testing, adhering to best practices is not just about academic rigor; it’s about ensuring that the tests conducted provide meaningful, actionable insights. This section aims to crystallize the significance of these best practices, focusing on aspects such as clarity in experimentation, understanding what matters, and being aware of common missteps.
Identifying Tests that Matter
One of the cornerstones of effective A/B testing is identifying which tests truly matter. The process isn't merely about testing for the sake of testing. Instead, it requires a keen eye for variables that could significantly impact user behavior and outcomes. For instance, let’s say an e-commerce website is pondering whether to change the color of the checkout button from green to red. This is a specific question that deserves investigation, especially if the user flow shows drop-offs at the checkout stage.
Before embarking on an A/B test, it’s essential to ask, "Does this change have the potential to influence key metrics?" Knowing where to focus your efforts can lead to substantial gains without unnecessary expenditure of resources.
Avoiding Common Pitfalls
Overtesting and Testing Too Many Variables
One prevalent challenge that marketers face is overt testing, which is essentially the practice of running multiple test variations concurrently. This approach can lead to muddled results. Consider a scenario: if a website is testing three different headlines and changing the layout at the same time, it becomes nearly impossible to ascertain which specific change influenced user behavior. Thus, testing too many variables can skew the data.
The key characteristic here is the reduction of clarity when interpreting results. Overt testing often leads to competing hypotheses that can obfuscate the true cause of any observed changes. Following best practices would involve isolating variables – test one thing at a time. This focused approach helps in simplifying interpretations, which ultimately yields more reliable insights.
Misinterpretation of Results
Misinterpretation of results is another pitfall that can derail the effectiveness of A/B testing. What may seem like a significant increase in conversions might simply be a result of random variances rather than a real change caused by the test. The key characteristic here is the lure of causation.
Often, marketers might fall into the trap of thinking they’ve identified a winning variant because the numbers seem favorable at first glance. However, this could be misleading without a robust understanding of statistical significance and confidence intervals. Misinterpretation can lead to poor decision-making and ultimately, a negative impact on business outcomes. Staying well versed in statistical fundamentals is central to countering this issue.
Iterative Testing Approach
An iterative testing approach fosters a cycle of continual improvement. After each A/B test, a comprehensive analysis should follow, drawing on the lessons of both successful and unsuccessful tests. Using these insights to guide further hypotheses and tests creates a feedback loop that enhances understanding and adaptation.
The foundation of this approach is rooted in flexibility. Markets evolve, user preferences shift, and what worked yesterday might not hold today. Consequently, adopting an iterative mindset turns A/B testing into a progressive tool rather than a one-off task. Each test informs the next, and over time, this leads to a more nuanced understanding of user behavior.
Beyond A/B Testing: Multivariate Testing
In the landscape of data-driven decision-making, A/B testing often takes center stage. However, there exists a nuanced approach that allows for more comprehensive exploration of user behavior: multivariate testing. This method builds on the principles of A/B testing but dives deeper, enabling businesses to assess multiple variables simultaneously. The significance of multivariate testing lies not just in its ability to test many factors at once, but in how it can provide richer insights and lead to more informed decisions.
Differences from A/B Testing
A/B testing primarily focuses on comparing two versions of a single variable to determine which performs better. For example, a website may compare two different headlines to see which attracts more clicks. In contrast, multivariate testing examines multiple variables and their interactions. Rather than just one headline, one might test different headlines, images, and call-to-action buttons all at once.
Here’s a quick comparison to illustrate:
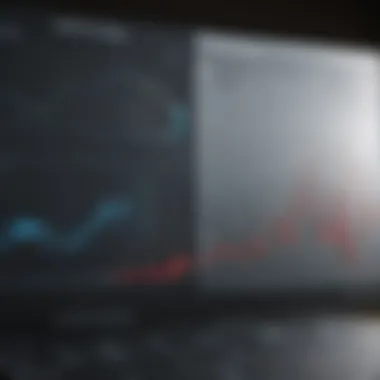
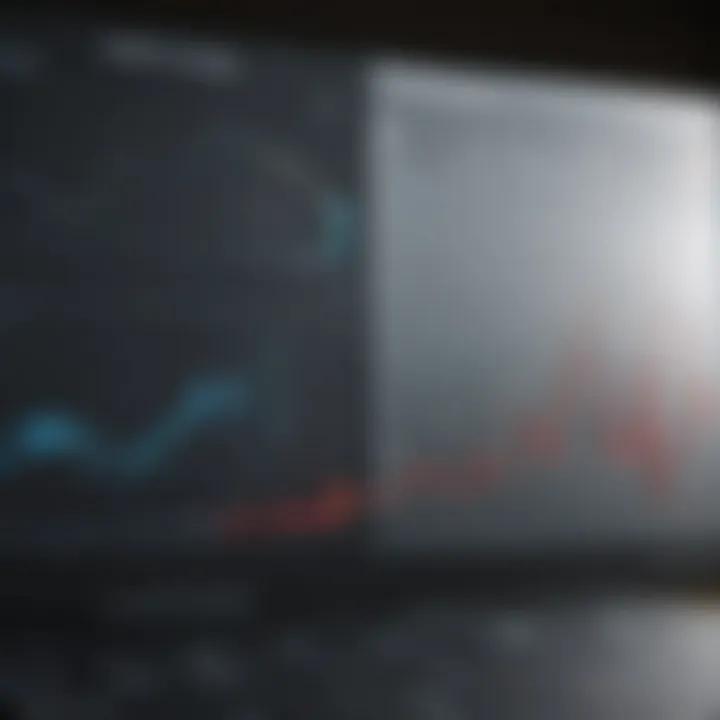
- A/B Testing: Tests one variable against another (e.g., headline A vs. headline B).
- Multivariate Testing: Tests multiple variables in conjunction (e.g., headline A with image A, headline A with image B, headline B with image A, and headline B with image B).
This complexity allows multivariate testing to identify which combination of variables works best while considering those interactions. In that way, marketers can glean insights into more dynamic, multifaceted user engagement and preferences. However, this too comes with a complex trade-off, requiring a larger sample size for valid statistical results, which can be a barrier for smaller businesses.
When to Use Multivariate Testing
Multivariate testing certainly has its place, though it’s not always the right approach. Here are some key scenarios where you might consider employing this method:
- Multiple Variables Are Present: If you have several elements on a landing page and want to test different combinations, multivariate testing can help. Imagine a site that has multiple banners, buttons, and text areas all vying for user attention; examining how they work together can yield pivotal insights.
- When You Have Sufficient Traffic: Multivariate testing demands a larger audience size than A/B testing, which requires careful planning. If your platform can pull in significant visitors, you’re in a prime position to take advantage of multivariate strategies.
- To Uncover Interaction Effects: Sometimes, it’s not enough to know that one variable performs better. Understanding how different elements interact can provide deeper insights. If you suspect that a specific combination could create a synergistic effect, multivariate testing is the way to go.
- For Refining Marketing Campaigns: When launching new marketing strategies, using multivariate testing can optimize ad copy and design to see what resonates best with your demographic.
Applications of A/B Testing in Various Fields
A/B testing isn't just a trendy buzzword floating around in marketing and development circles; it’s a powerful tool that opens doors to data-driven decision-making across different fields. Each area of application reveals not just the versatility of A/B testing but also its critical role in shaping user experiences and improving outcomes. Important benefits include the ability to make informed changes, optimize strategies, and ultimately drive growth based on real user interactions. The beauty of A/B testing lies in its simplicity—comparing two versions to see which performs better—yet the implications of its findings can be profound.
E-commerce Optimization
In the realm of e-commerce, the stakes are high. An optimized website can drive up conversion rates and increase revenue. Here, A/B testing shines by allowing businesses to experiment with various elements, such as website layouts, product images, and pricing strategies. For instance, a clothing retailer might test two different homepage designs: one featuring seasonal promotions and the other showcasing best-selling items. By analyzing metrics like bounce rates and time spent on the site, the retailer can determine which design resonates more with its audience.
Key elements to consider in e-commerce A/B testing include:
- Product Page Layout: Changing the arrangement of images and text can influence customer decisions significantly.
- Call-to-Action Buttons: Testing color, text, and placement can affect click-through rates.
- Checkout Process: A/B testing different steps in the checkout process can uncover barriers reducing cart abandonment.
"In e-commerce, tiny tweaks can mean the difference between a sale and a lost opportunity. Don’t underestimate the power of A/B testing."
Email Marketing Strategies
Email marketing remains a staple in many businesses' marketing arsenals. A/B testing brings significant advantages, allowing marketers to refine their strategies with precision. For instance, subject lines can make a huge difference in open rates. A business might experiment with two subject lines—one direct and one catchy—to see which garners more interest.
Noteworthy factors to test include:
- Sending Times: Discovering the optimal day and time can dramatically boost engagement.
- Content Layout: Comparing different formats (text-heavy vs. image-heavy) helps identify audience preferences.
- Personalization: Testing personalized emails versus generic ones can provide insights into what resonates better with recipients.
When done right, each A/B test serves as a stepping stone to discovering what maximizes engagement and conversion rates in email campaigns.
Web and App Development
For developers, A/B testing serves as a fundamental component in creating user-friendly applications and websites. Whether it's tweaking the user interface or modifying functionality, the insights gained from small tests can lead to more satisfying user experiences. Developers can deploy an application efficiency test, where one version features a simplified navigation menu while another retains a more complex design. Analyzing user interaction data helps determine which layout enhances user engagement more effectively.
Essential considerations include:
- User Interface Changes: Testing different UI elements helps in creating a more intuitive experience.
- Feature Usability: Features can be trialed on a portion of users to identify their impact on overall satisfaction.
- Loading Speeds: Comparing various strategies for speeding up page loads can directly affect user retention.
In essence, A/B testing in web and app development can guide developers to make informed, user-centric changes that lead to better products and happier users.
Concluding Insights on A/B Testing
A/B testing stands as a cornerstone in the realm of data-driven decision making. It encapsulates a method that not only drives optimization but also fosters innovation. Engaging in this practice reveals insights that might otherwise remain hidden. By directly comparing two versions of the same variable, organizations can make informed choices that ultimately enhance user engagement and satisfaction.
The Future of A/B Testing
As we gaze into the horizon, the evolution of A/B testing is bound to intertwine with advancements in technology and data analytics. The integration of artificial intelligence and machine learning is paving the way for more sophisticated testing methodologies. These technologies will likely enable testers to automatically generate hypotheses based on user behavior, leading to a more streamlined testing process. For instance, instead of manually selecting which variables to test, an AI might analyze historical data and suggest test variations that have a higher probability of success.
Moreover, as personalization becomes an ever-increasing priority for businesses, A/B testing will adapt accordingly. The challenge will be to cater to an audience that is not only diverse but continually evolving. Companies will need robust frameworks capable of handling these complex needs, leading to a push for more granular testing approaches, possibly expanding into territory like dynamic content adaptation on websites or apps.
"The future of A/B testing isn't just about A vs. B, but about understanding the vast spectrum of user preferences and needs."
Encouragement for Continued Learning
Understanding A/B testing is not a one-and-done process. It requires a commitment to continuous learning. New methodologies emerge, accompanied by fresh insights and best practices. Engaging with communities and resources, such as forums on Reddit or statistics courses on educational platforms, can sharpen one's skills significantly.
To that end, the importance of keeping abreast of developments in statistical analysis, A/B testing tools, and user experience design cannot be overstated. Workshops and webinars can serve as invaluable resources, where practitioners share real-world challenges and solutions they encountered during testing.
- Consider subscribing to industry journals or attending conferences on marketing analytics to expand your knowledge base.
- Network with fellow marketers through platforms like Facebook groups dedicated to A/B testing and optimization strategies
Ultimately, staying curious and being willing to adapt is what keeps the field vibrant and effective. The more one understands the nuances of A/B testing, the better equipped they are to utilize this powerful tool in driving meaningful business outcomes.