Exploring Graphing Techniques for Data Visualization
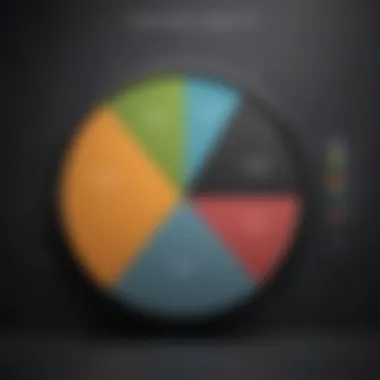
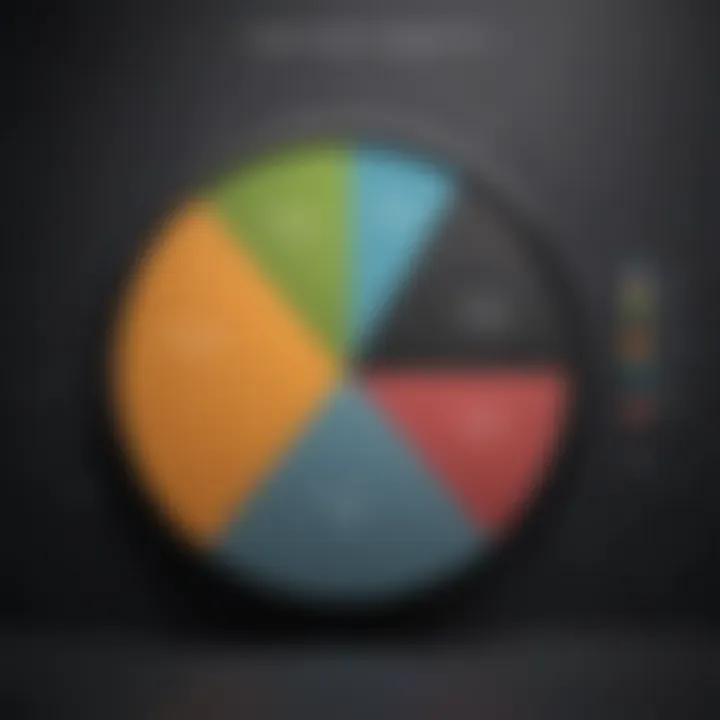
Intro
Data visualization is the heartbeat of understanding complex information. In todayβs fast-paced financial arenas, how data is presented can make all the difference between a sound investment decision and a costly mistake. Grasping diverse graphing techniques is essential for anyone aiming to make clued-up choices in investments. The application of various graphsβfrom line charts to pie graphs or histogramsβoffers unique insights and can effectively highlight trends, growth patterns, or anomalies.
This guide promises to dig deep into the nitty-gritty of these graphing techniques and their real-world applications. With a focus on financial contexts, weβll traverse through the distinct characteristics of several graph types, helping you discern which method best communicates your dataβs story. Letβs roll up our sleeves and dive into the world of investment terminology to set a solid foundation for our discussion.
Investment Terminology
Understanding the language of investing is crucial. Before taking on graphing techniques, itβs wise to familiarize yourself with the key terms and concepts that populate this field. Knowing the vocab isnβt just academic; itβs practical. The clarity in communication can guide analytical thinking and decision-making.
Key Terms and Definitions
- Equity: Ownership in a company. Owning shares means holding a piece of that business.
- Dividend: A portion of earnings distributed to shareholders, often seen in investment strategies focused on income.
- Market Capitalization: The total value of a company's outstanding shares. It gives investors a quick look at the company's size.
- Volatility: The rate at which the price of a security increases or decreases for a given set of returns. High volatility can mean higher risk.
Common Acronyms in Finance
- EPS: Earnings Per Share β a key measure of a company's profitability.
- ROI: Return on Investment β a formula to evaluate the efficiency of an investment.
- P/E Ratio: Price to Earnings Ratio β a valuation ratio of a company's current share price compared to its per-share earnings.
"Understanding financial terms and their implications is not just about jargon; itβs about seeing the bigger picture and making astute decisions based on data."
Expert Insights
Equipped with the necessary terminology, now we can explore investment strategies and gain some tips from seasoned professionals.
Investment Strategies Overview
Diverse investment strategies can be likened to a toolkitβvarious methods for different tasks. For instance:
- Value Investing: Buying undervalued stocks that have a potential for growth.
- Growth Investing: Focusing on companies expected to grow at an above-average rate.
- Income Investing: Concentrating on generating regular income through dividends and interest.
Tips from Financial Advisors
Experts have a treasure trove of wisdom to impart:
- Start with a Clear Goal: Define what you seek from your investments. Is it short-term gain or long-term stability?
- Stay Informed: Follow market trends and news to make timely decisions. Knowledge is power in the financial world.
- Diversify Your Portfolio: Donβt put all your eggs in one basket. A mix of assets can help manage risk much better.
These fundamental insights lay a sturdy groundwork as we continue to navigate through the myriad options in graphing techniques. Next, weβll shift gears to analyze the specific graph types available and their applications in elucidating financial data. Stay tuned.
Types of Graphs: An Overview
Graphs serve as visual storytelling tools, essential in any data-driven environment. With the right type of graph, complex data can become understandable, transforming raw numbers into insightful narratives. This article explores the various types of graphs available, the importance of each category, and the implications they have in financial analysis and other sectors.
Definition and Importance of Graphing
Graphing, at its core, is converting numerical data into a visual format that highlights relationships, trends, and patterns. This transformation plays a pivotal role in our comprehension of data, particularly in the realm of finance, where investors often face an avalanche of figures. By employing graphs, investors can absorb large amounts of information quickly, allowing for faster decision-making processes. In financial contexts, a well-chosen graph clarifies trends, enhances analysis, and supports strategic choices.
For instance, a line graph might clearly illustrate the ups and downs of a stock price over time, enabling investors to easily identify patterns or anomalies. On the flip side, presenting the same data as a table could lead to missed insights.
"A picture is worth a thousand words. In finance, it can be worth a thousand dollars too."
Historical Context of Graphing Techniques
The practice of graphing has deep roots, tracing back centuries. The earliest forms of graphs were used by mathematicians like RenΓ© Descartes and Sir Isaac Newton. These early graphs served as tools for calculating and visualizing mathematical equations and scientific phenomena. Fast forward to the 18th and 19th centuries, and we see a blossoming of statistical graphs pioneered by figures like William Playfair, who created bar and pie charts to convey economic data.
Over time, graphing has evolved with technology, leading to increasingly sophisticated techniques that allow for multi-dimensional representations of data. Today, advanced software enables real-time data visualizations, breathing life into historical trends and predictions. In essence, understanding the historical context of graphing not only enhances our appreciation of the craft but also informs our current applications and methodologies.
Through this exploration of graph types, their importance, and their storied past, investors and financial enthusiasts can grasp better how to leverage graphs in their analyses and reports.
Understanding the fundamentals is the first step toward utilizing these tools effectively in the pursuit of informed decision-making. This understanding lays the groundwork for diving deeper into the specific types of graphs and their numerous applications.
Bar Graphs: Visualizing Categorical Data
Bar graphs stand as a cornerstone in the world of data visualization, particularly when we're dealing with categorical data. Their straightforward format provides an immediate visual impression of comparisons across various categories. Imagine needing to convey how sales figures stack up against one another for different products. A bar graph transforms numbers into visuals that are easily digestible at a glance. This visual tool isn't just an aesthetic choice; it plays a crucial role in comprehension and interpretation of data, making it an indispensable ally for professionals in various domains.
Characteristics of Bar Graphs
Bar graphs are characterized by a series of rectangular bars illustrated either vertically or horizontally. Each bar's length is proportional to the value it represents. Typically, the categories are placed along one axis (usually the x-axis for vertical bars) while the values are plotted along the other (y-axis).
- Clear Comparisons: The distinct separation of bars allows for easy comparison between categories. The eye naturally gravitates towards differences in height or length, making this format effective for highlighting disparities.
- Categorical Grouping: This graph type can efficiently display multiple groups without overcrowding the visuals. For example, it can clearly show how different departments within a company are performing during a fiscal quarter.
- Variable Width: Though bars are often uniform in width, they can vary to convey additional information (such as volume or frequency) adding another layer to their interpretation.
- Color Coding: Bar graphs frequently make use of color to distinguish categories, enhancing readability and engagement. For example, in a financial report, one could use green for profits and red for losses, allowing for instant recognition of trends.
Applications in Financial Analysis
In the world of finance, bar graphs find their footing in various contexts, providing both clarity and depth. These are some specific applications where bar graphs shine:
- Sales Analysis: They can represent sales figures across multiple product lines, enabling businesses to pinpoint which items are doing well and which arenβt. By simply glancing at the heights of the bars, one can derive insights about market performance.
- Budget Allocation: Companies often utilize bar graphs to visualize budget allocations across departments. It allows stakeholders to see how resources are distributed, highlighting potential areas for optimization.
- Expense Tracking: Bar graphs can track different categories of expenses, such as operational costs versus marketing spends. This aids in identifying where most money flows and how it aligns with goals.
- Comparative Performance: When examining the financial health of different companies or sectors, a bar graph facilitates straightforward comparisons, allowing investors and analysts to quickly ascertain which players in the market are thriving.
- Historical Trends: By displaying annual revenues across years as bars, financial analysts can interpret growth patterns. A bar graph can effectively portray how a company's earnings have surged or dipped over particular periods.
Ultimately, bar graphs serve as a practical and versatile tool in financial analysis. Their ability to distill complex data into simple visual representations remains invaluable for both novice and experienced investors. They make number-crunching not just straightforward, but visually appealing and markedly informative.
Line Graphs: Tracking Changes Over Time
Line graphs serve as key tools in the world of data visualization, especially when it comes to tracking changes over time. They provide a visual representation that allows investors and analysts to observe trends, patterns, and fluctuations in data sets at a glance. The clarity and effectiveness of line graphs make them highly relevant for individuals looking to make informed decisions based on historical performance, be it in finance, sales, or other metrics where time plays a critical role.
Features of Line Graphs
At their core, line graphs consist of a series of data points connected by straight lines. Some notable features include:
- Axes: The horizontal axis (x-axis) typically represents time intervals, while the vertical axis (y-axis) illustrates the values of the data being analyzed. This two-dimensional layout emphasizes the relationship between the two variables.
- Data Points: These represent individual observations recorded at specific time intervals. Each point on the graph signifies a data entry, making it easy to discern how values shift over time.
- Line Connecting: The lines that connect the data points guide the eye through the progression and trends, effectively showing rises and falls in value.
- Multiple Lines: Often, multiple lines can appear on the same graph, allowing for side-by-side comparisons of different datasetsβthis is particularly useful in financial analysis to compare performance metrics across different assets or firms.
"A line graph can tell a story that tables simply can't; it shows the flow, the changes, and often the underlying dynamics affecting the data."
Use Cases in Investment Trends
When it comes to investment trends, line graphs are simply indispensable. Their applications in the financial sphere include:
- Monitoring Stock Prices: Investors utilize line graphs to track stock price movements over time. This helps in identifying trends like bullish or bearish markets, allowing for timely decision-making.
- Portfolio Performance: Individuals managing investments can represent their portfolio growth over certain time periods, aiding in understanding whether their investment choices resonated with market trends.
- Economic Indicators: Economists and analysts can utilize line graphs to visualize essential economic indicators such as inflation rates or unemployment rates over time. Observing these trends can provide insights for policy-making and business strategies.
- Sales Growth Analysis: For businesses, tracking sales performance over time through line graphs reveals whether marketing efforts are yielding positive results or if there are declines that need addressing.
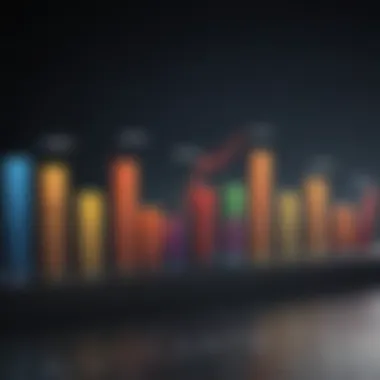
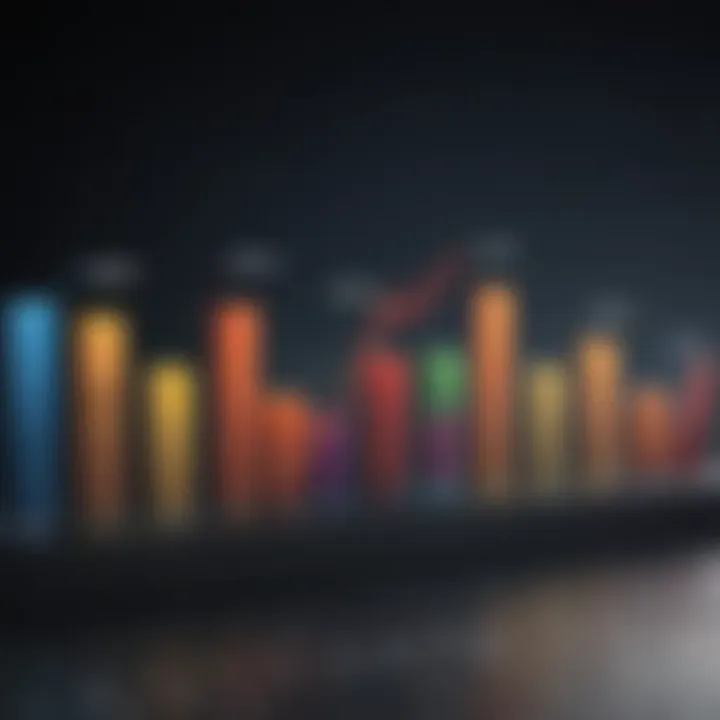
In summary, line graphs are more than just a simple visual; they act as powerful instruments for comprehending historical trends. They facilitate analytical thinking and strategic decision-making, supporting investors to navigate the often unpredictable financial waters.
Pie Charts: Representing Proportions
Pie charts have taken their place as a widely recognized tool for representing data in a visually appealing manner. They serve to break down complex information into digestible segments, allowing quick comprehension of proportions. This effectiveness makes pie charts an important component in the arsenal of graphing techniques, especially within financial analysis where stakeholders often seek to understand shares and distributions at a glance.
Understanding Pie Charts
A pie chart is composed of a circular graph divided into slices, each representing a proportion of the whole. The total area of the pie reflects 100%, with each slice denoting different categories within that total. This type of graphical representation is particularly effective when illustrating parts of a wholeβlike market shares or budget distributions.
Here are some essential features of pie charts:
- Visual Clarity: The circular nature makes it easy to compare relative sizes of categories. The larger the slice, the greater the proportion.
- Simplicity: They convey information without overwhelming the viewer, making them suitable even for audiences unfamiliar with data interpretation.
- Immediate Insight: When done right, pie charts deliver instant clarity about proportions, helping to drive discussions or decisions based on visual evidence.
However, potential drawbacks exist. Pie charts can become cluttered and hard to interpret if too many slices are used. It's advised to limit categories to maintain clarity. Too many slices can lead to confusion rather than enlightenment.
When to Utilize Pie Charts
Determining when to use pie charts involves understanding the kind of data you have. Here are key considerations for their effective application:
- Limited Categories: Use pie charts when you aim to display data with just a few segments. Typically, four to six slices are manageable and concise. For instance, showing market share among a few companies can be impactful.
- Emphasis on Proportions: If your goal is to highlight how parts contribute to a whole, pie charts shine. They clearly indicate which segments dominate or how evenly distributed certain characteristics are.
- Immediate Comparisons: When quick comparisons among sections are necessary, pie charts can give an at-a-glance perspective that saves time.
Pie charts are ideal in scenarios like:
- Portfolio distributions in an investment context, where different asset classes are displayed as parts of a whole.
- Survey results breaking down responses into categories, such as customer satisfaction levels.
An important note: accuracy is crucial. While pie charts are eye-catching, any misleading proportions can skew interpretations, leading to incorrect assumptions. Thus, keep the data truthful and always aim for clear labeling.
"A good pie chart doesn't just show data; it tells a story visually. The best pie charts engage the viewer and make complex information clear."
In summary, pie charts are powerful tools for representing proportions when used prudently. They pave the way for effective communication of data nuances, especially when clarity is paramount. As one learns to navigate through various datas, mastering the art of pie chart construction can become a vital skill.
Scatter Plots: Analyzing Relationships
Scatter plots serve as a dynamic tool in the realm of data visualization, crucial for unpacking the intricate relationships between variables. By plotting data points on a two-dimensional graphβwhere each axis corresponds to a different variableβscatter plots facilitate an immediate visual interpretation of potential correlations. Unlike other graphing techniques, scatter plots allow analysts to detect patterns or clusters that may be less discernible in other forms of data representation. This section aims to elucidate the foundational principles governing scatter plots and the significance of identifying correlations in a variety of applications, especially in financial analysis.
Principles of Scatter Plots
At their core, scatter plots adhere to a few simple yet critical principles:
- Two Variable Analysis: Each point on the scatter plot corresponds to an entry in a dataset, with one variable on the x-axis and another on the y-axis. This duality enables a nuanced exploration of how the two variables interact with one another.
- Visual Patterns: The arrangement of data points can reveal trends. A tight cluster of points might suggest a strong correlation, whereas a more dispersed pattern may indicate a weaker relationship. Identifying these patterns is integral to interpreting the significance of the data.
- Outliers: Scatter plots can highlight outliersβdata points that deviate from the overall pattern. Recognizing these anomalies can be pivotal in financial contexts, where extreme values might reflect unusual market behavior or errors in data reporting.
In practice, to construct an effective scatter plot:
- Select Data: Choose two variables that you suspect may have a relationship.
- Plot Points: Each point should be plotted based on its corresponding values for the x and y variables.
- Analyze the Visuals: Look for clusters, linear patterns, or any signs of correlation. This initial visual examination can guide further statistical analysis.
Identifying Correlations in Data
Identifying correlations through scatter plots can bring considerable clarity to data analysis, especially in investment scenarios where understanding relationships is paramount. Here are key aspects to consider:
- Types of Correlation: Understanding the types of correlation is essential. Positive correlation signifies that as one variable increases, the other tends to also increase, while negative correlation indicates that as one variable ascends, the other declines.
- Quantifying Correlation: A visual assessment is often paired with statistical methods, such as calculating the correlation coefficient (r-value), which quantifies the strength and direction of a relationship. This coefficient can range from -1 to 1, with values closer to 1 indicating a strong positive correlation and values nearing -1 reflecting a strong negative correlation.
- Practical Applications: In the context of financial analysis, recognizing correlations can inform investment strategies. For instance, if a scatter plot reveals a strong positive correlation between the price of oil and airline stocks, investors might choose to adjust their portfolios based on shifts in oil prices.
"Correlations can guide investors in navigating complex markets; knowing what moves together can be the key to making informed decisions."
Histograms: Frequency Distribution Visualization
Histograms play a significant role in the realm of data visualization, especially when it comes to illustrating the distribution of data points across a range. This technique allows for a clear representation of frequency distribution, making it easier for analysts and decision-makers to grasp how their data is spread out. Because of their ability to reveal patterns and trends within large datasets, histograms are indispensable in various fields, particularly in finance, where they can provide insights into market behavior and risk evaluation. By highlighting the frequency of occurrences within specific ranges, histograms empower investors to make informed decisions based on historical data and anticipated trends.
Creating and Interpreting Histograms
The creation of a histogram entails several essential steps. First, data needs to be organized into intervals, commonly referred to as bins. These bins represent ranges of values, and the width of each bin can significantly affect the resulting graph. A narrow bin width may yield a more detailed view, while a wider bin can smooth out fluctuations and highlight general trends.
Hereβs a simple way to create a histogram:
- Collect Data: Gather your dataset, ensuring it is relevant to your analysis.
- Determine the Range: Identify the minimum and maximum values within your dataset to establish the overall range.
- Setup Bins: Decide on the size and number of bins to appropriately categorize your data. A common rule is to start with about five to ten bins, adjusting as needed.
- Tally Frequencies: Count how many data points fall into each bin and record this frequency.
- Draw the Histogram: Use graphing software or tools to plot your bins on the x-axis and the corresponding frequencies on the y-axis.
Once the histogram is created, interpreting its features becomes pivotal. Peaks in the histogram suggest areas where data points cluster, while the width and height of the bars indicate trends and the distribution's skewness. A right-skewed histogram may hint that most values are lower, with a few higher outliers, while a left-skewed one suggests the opposite.
Application in Risk Assessment
In the context of finance, histograms serve as a powerful tool for risk assessment and management. By representing historical returns or market movements, analysts can better visualize volatility and identify patterns that may impact future performance. For instance, when assessing the risk of an investment portfolio, a histogram can demonstrate the frequency of returns falling within different ranges, allowing investors to understand potential outcomes and their probabilities.
βIn risk management, understanding the distribution of returns is crucial to minimize potential losses.β
A few key uses of histograms in risk assessment include:
- Visualizing Historical Performance: By showing past performance frequency, histograms help investors gauge how often an asset has met or exceeded certain thresholds.
- Understanding Value at Risk (VaR): This metric quantifies the potential loss in value of an asset or portfolio under normal market conditions. Histograms can illustrate how often returns fall below a specific value, aiding in calculating VaR.
- Volatility Analysis: A histogram can reveal the volatility of returns, with wider distributions indicating more risk and potential price swings.
In summary, histograms offer a straightforward but effective way to visualize data distributions, particularly in risk assessment scenarios. Their ability to highlight trends and frequency distributions can greatly assist both novice and experienced investors in making informed decisions.
Whether itβs assessing market volatility or analyzing investment returns, the value of histograms in data-driven decision-making is undeniable.
Box Plots: Summarizing Data Characteristics
In the realm of data visualization, box plots stand as a robust tool for summarizing complex datasets succinctly. They offer a visual representation of key statistical measures, allowing one to easily grasp the distribution, variability, and potential outliers within the data. This type of graph is particularly valuable in financial applications, where quick insights into data characteristics can guide decision-making and risk management.
The importance of box plots lies in their ability to convey a multitude of information at a glance. For investors and analysts, understanding the central tendency and spread of data is crucial. Box plots provide an effective way to see the median, quartiles, and extremes of a dataset, thereby enhancing analytical capabilities.
Moreover, by using box plots, one can easily compare different groups or categories of data. This feature makes them particularly useful when analyzing performance metrics across various investment categories or assessing returns from different financial instruments.
Understanding Box Plot Components
A box plot might seem simple at first glance, but it comprises several critical components that contribute to its effectiveness:
- Minimum and Maximum Values: These points represent the lowest and highest values in the dataset, excluding outliers. They provide a frame of reference for the overall range of data.
- Lower Quartile (Q1): This is the median of the lower half of the dataset, showing the point at which 25% of the data fall below.
- Median (Q2): The central value that divides the dataset into two equal halves. This is a crucial indicator of the dataset's central tendency.
- Upper Quartile (Q3): This reflects the median of the upper half, indicating where 75% of the data fall below.
- Interquartile Range (IQR): Defined as the difference between Q3 and Q1, the IQR measures the middle 50% of the data and is vital for understanding data dispersion.
- Outliers: These points, often plotted as separate dots outside the whiskers, indicate data that deviates significantly from the rest. Recognizing outliers can lead to deeper analysis and investigation into their causes.
Usage in Performance Comparison
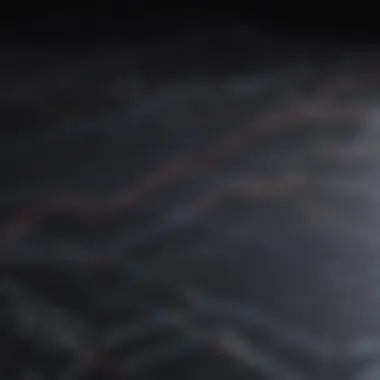
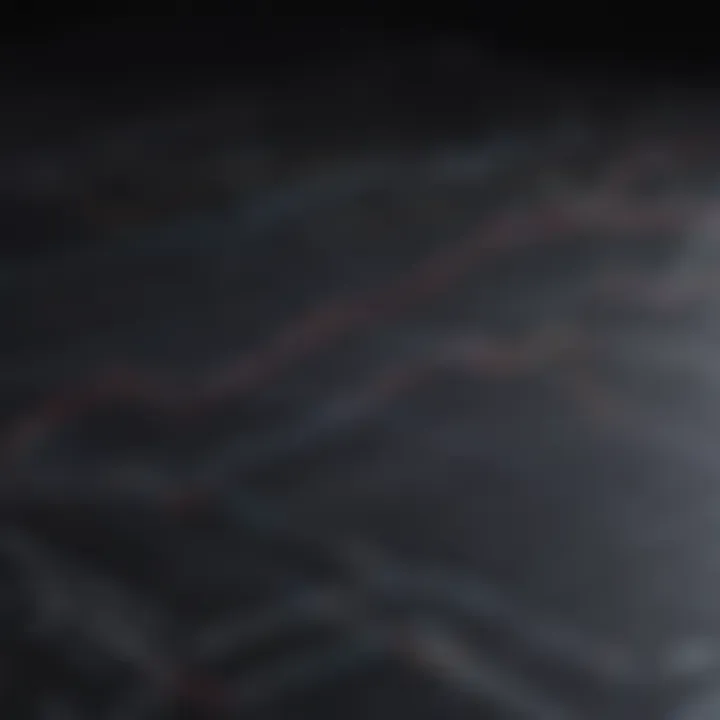
Box plots serve as a powerful means of comparing performances across various variables or categories. For instance, an investor can utilize box plots to analyze the performance of different stocks or funds over a specified time frame. This visual comparison quickly highlights significant differences in returns, volatility, and risk associated with each choice.
To make it clearer, consider the following applications:
- Identifying Trends: By comparing box plots of data from different periods, one can easily observe trends in performance, helping to inform future investment strategies.
- Risk Assessment: In finance, understanding the range and variability of asset returns is crucial. Box plots facilitate an assessment of risk by showcasing how closely or widely performance results cluster around the median.
- Decision-Making: When faced with multiple investment options, visualizing the performance data through box plots can clarify which options hold a more favorable risk-return profile.
"Box plots provide an astute glimpse into data distributions, making complex data accessible for quick analysis and comparisons."
The value box plots bring to the table cannot be overlooked. For novice and seasoned investors alike, mastering the use of box plots enhances not just analytical skills but also overall investment success.
Heat Maps: Visualizing Data Density
Heat maps are powerful tools in the realm of data visualization, providing a clear and insightful way to represent complex information. These graphical representations allow investors and analysts to see patterns and trends that may be hidden in numerical data. The virtue of a heat map lies in its ability to transform seemingly overwhelming data sets into a visually digestible format, often revealing correlations or outliers that are critical in decision-making processes.
One of the prime advantages of heat maps is their intuitive nature. They use color gradients to signify the magnitude of data, making it easier to pinpoint where densities lie. Warm colors commonly indicate high values, while cooler shades suggest low values. This visual cueing allows even those new to data analysis to grasp essential insights without sifting through stacks of numbers.
"Heat maps are like the lighthouse in the fog of data; they guide you to see the light in your information."
Attributes of Heat Maps
Heat maps possess distinct characteristics that enhance their utility:
- Color Encoding: The use of color gradients is foundational to heat maps, with different hues representing varying data density. This intuitive color coding speeds up the comprehension of complex information.
- Gradient Representation: By utilizing gradients, heat maps convey a range of values seamlessly. Instead of discrete values, users can appreciate subtle changes in data trends through a spectrum of colors.
- Scalability: Heat maps are versatile in scale, suitable for both small data sets and extensive databases. This scalability makes them particularly appealing in diverse fields, from finance to healthcare.
- Interactivity: Many modern heat maps allow for user interaction. Analysts can hover over different segments to obtain precise data points, fostering a deeper understanding of trends without overwhelming the viewer with information.
These attributes, when harnessed properly, can produce exceptional clarity in data representation, especially for multi-dimensional data sets that could otherwise feel insurmountable.
Applications in Market Analysis
Heat maps find significant application in market analysis, primarily for the following reasons:
- Identifying Market Trends: Investors can use heat maps to analyze sales data across different geographies. By looking at heat maps of sales performance, one can quickly see which regions or products are yielding the best returns, facilitating effective market strategy adjustments.
- Real Estate Insights: The real estate market benefits tremendously from heat maps, allowing investors to visualize property values or growth potential across zip codes or neighborhoods. Areas heating up can be apparent just from the color intensity on the map.
- Customer Behavior Mapping: Retail analysts often employ heat maps to understand customer interactions throughout a store or online. By analyzing where customers linger, one can optimize store layouts or adjust marketing strategies.
- Risk Assessment: In volatile market situations, heat maps can visualize risk levels across different sectors or assets, helping investors make informed, timely decisions to mitigate potential losses.
Bubble Charts: Depth in Data Representations
Bubble charts offer a unique layer of depth when it comes to data representation. They enable the visualization of relationships among three or more variables at once, which is something many other graph types struggle to accomplish. The size of each bubble indicates a specific variable's value, while its position on the X and Y axes demonstrates the correlation between two variables. This ability to convey multiple dimensions of data in one single view can be particularly beneficial for investors looking to analyze complex financial situations or market trends.
Structure of Bubble Charts
A bubble chart is essentially a scatter plot where each point is replaced by a circle (the bubble). Here are the key components of a bubble chart:
- X-Axis and Y-Axis: The horizontal and vertical axes represent two distinct variables. For instance, an investor might plot time on the X-axis while venture capital funding is shown on the Y-axis.
- Bubble Size: The diameter of each bubble signifies the value of a third variable. For example, in market analysis, a company's yearly revenue could dictate the bubble's size, giving immediate visual context about its scale in comparison to competitors.
- Color Coding: Bubbles can also utilize color to indicate categories or qualitative characteristics. For example, different sectors within the same chart can be color-coded to differentiate between technology, healthcare, and finance.
Combining these elements allows analysts to extract significant insights at a glance. Too often, investors drown in data, but a well-structured bubble chart can cut through that noise and highlight critical points.
Usage in Multidimensional Data Analysis
The applications of bubble charts extend across various dimensions in data analysis. Hereβs how theyβre typically deployed:
- Market Research: Investors can utilize these charts to analyze various companies based on sales performance, growth rates, and market size. This multidimensional view can reveal patterns that may not be apparent in simpler charts.
- Investment Portfolios: By representing different assets in a bubble chart, investors can assess risk versus return for various investments. For example, one can compare high-risk startups against established firms, evaluating both the potential return and associated risks through size and position.
- Comparative Analysis: These charts allow for straightforward comparisons between entities. For example, an investor might want to see the relationship between marketing budget and revenue across different companies; bubble charts invite clear visual differentiation.
Insightful Note: When interpreting bubble charts, it's crucial to remain mindful of context. The size of the bubbles can drastically change how we perceive importance or risk.
Radar Charts: Comprehensive Comparison of Variables
Radar charts, also known as spider charts or polar charts, stand as a powerful tool for comparing multiple variables. They provide a visual representation that allows quick and easy analysis of complex datasets. In financial contexts, where different metrics and categories must be assessed simultaneously, radar charts prove invaluable. With their unique structure, they help in identifying trends and areas needing attention, making them crucial for performance evaluation and comparisons.
Key Features of Radar Charts
Radar charts come with several defining characteristics that make them distinctive:
- Multidimensionality: The star-like shape enables the plotting of multiple variables on axes radiating from a center point. Each axis represents a different variable, allowing comparisons at a glance.
- Data Overlap: Multiple datasets can be represented on the same chart. This overlapping is useful for comparing different entities or timeframes without cluttering.
- Scale Representation: Each variable can have its own scale on respective axes, providing flexibility in representing diverse data types.
With these features, radar charts engage audiences by making complex relationships more comprehensible.
Situational Applications in Performance Analysis
Radar charts find their niche in various situations, particularly in performance analysis across different sectors. Here are some practical use cases:
- Employee Performance Evaluation: Businesses can use radar charts to assess employee performance on various competencies like communication, problem-solving, and teamwork.
- Product Comparisons: Companies can visually display the strengths and weaknesses of several products in relation to one another, helping consumers make informed decisions.
- Investment Portfolio Assessment: Investors might plot the attributes of various stocks or investment options against one another, enabling a clear view of diversification and risk metrics.
In the ever-evolving landscape of finance, radar charts help stakeholders tune into the multiple facets of data simultaneouslyβthat's why they can be a game changer.
In defense of their limited use, itβs useful to note that radar charts might become unwieldy if too many variables are compared at once, which can lead to interpretative confusion. Thus, moderation in the number of dataset variables is advised for clearer insights.
Area Graphs: Showcasing Volume Along Trends
Area graphs are a compelling tool in the domain of data visualization, especially when it comes to showcasing changes in volume over time. They effectively communicate data trends by emphasizing the magnitude of change. This can be particularly useful in financial analysis, where understanding fluctuations in specific metrics is crucial for making informed decisions. Area graphs not only provide a visual context for data but also help to amplify the narrative behind it by making larger trends apparent.
Defining Area Graphs
Area graphs combine elements of line graphs and bar graphs. At their core, they display quantitative data over a particular time frame. The key characteristic is the shaded area between the line and the baseline of the graphβthis area signifies cumulatively the value of the data points. Essentially, while the line offers a clear trajectory of data points related to the whole, the area beneath it elucidates the total volume. This visual representation can lead to a more intuitive understanding of how data evolves through different periods.
Another point worth considering is that area graphs can be used to compare multiple datasets within a single visual framework. By layering the areas, viewers can grasp not only how one data set fares against another but also track individual trends.
Visualizing Financial Growth and Decline
In the financial world, area graphs serve as invaluable tools for illustrating growth and decline. Investors often rely on these graphs to make educated guesses regarding future market movement. By showcasing trends over time, such as revenue, expenses, or stock price fluctuations, they enable a clearer assessment of an entity's financial trajectory.
When adopting area graphs for financial data, itβs vital to ensure clarity:
- Maintain a clean design: Overly complicated designs can muddle the intended message.
- Label axes clearly: This helps in accurate interpretation of the data.
- Choose appropriate color schemes: Colors should differentiate data sets without overwhelming the viewer.
For example, a technology firm might use an area graph to depict its revenue growth over several fiscal years. The graph could illustrate a steady increase from 2015 to 2019, followed by a significant dip in 2020 due to unexpected market conditions, before showing a rebound in 2021. Such visuals allow stakeholders to quickly comprehend the essence of the firm's performance and are instrumental in monitoring investment viability.
In summary, area graphs not only emphasize trends in financial growth and decline but also provide a holistic view that can help investors navigate complex data landscapes.
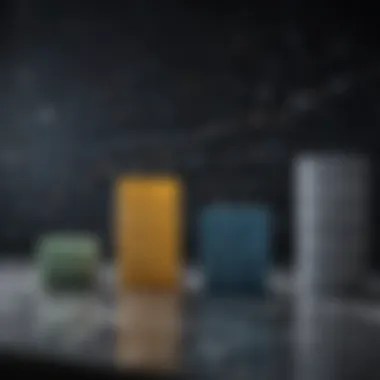
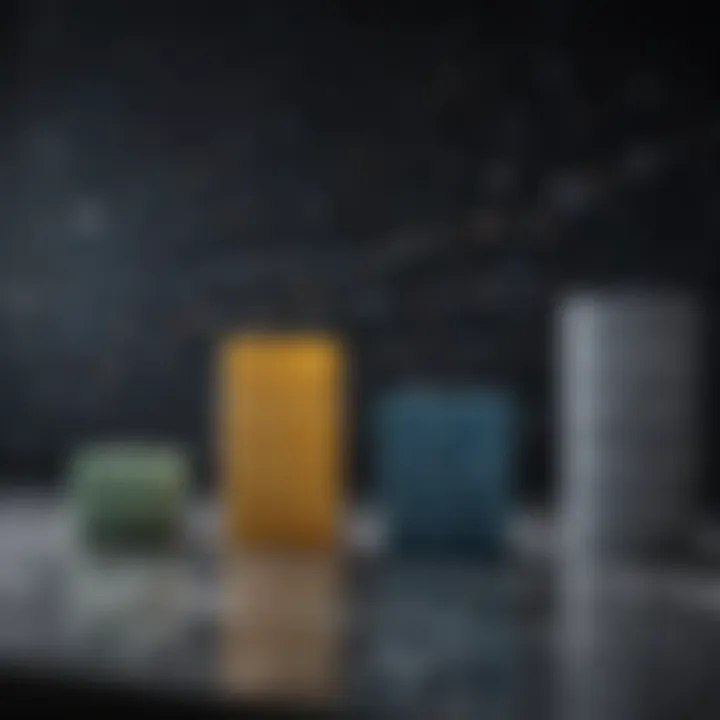
In the fast-paced world of finance, where every second counts, area graphs perform admirably to distill critical information into readily digestible formats, making them essential tools for both novice and experienced investors alike.
Network Diagrams: Mapping Relationships
In the realm of data visualization, network diagrams stand out for their ability to depict complex relationships and connections between various entities. These diagrams allow viewers to grasp intricate systems, showcasing how different components interlink, interact, and influence one another. The significance of network diagrams cannot be overstated, especially in a world brimming with interconnectivity. Businesses, researchers, and analysts leverage these tools to discern patterns, identify key players, and navigate elaborate webs of relationships efficiently.
Understanding Network Structures
Network structures are often composed of two fundamental parts: nodes and edges. The nodes represent the entities, be they people, organizations, or systems, while the edges symbolize the relationships or interactions between them.
By focusing on the connection type, such as communication, collaboration, or transactions, network diagrams can provide a clearer image of how certain individuals or entities relate to each other. This enables stakeholders to learn a lot about the dynamics at play in any given scenario. Visualizing these connections can unveil the underlying structure of networksβfrom tightly-knit groups to sprawling clusters where connections may be more diffuse.
Like a spiderβs web, the strength and type of connections can vary, revealing crucial insights. For example, in social networks, a node with several strong connections could be a pivotal influencer. Similarly, in business contexts, understanding what entities are connected and how helps organizations develop strategic partnerships or identify potential gaps in their approach.
Applications in Investment Networks
In the financial sector, network diagrams serve multiple purposes. One of the primary applications is in investment networks, where they illuminate connections between various investments, companies, and the market dynamics at play. Investors can use these diagrams to see how different industries interact and how the performance of one sector may influence another, or even entirely different markets.
By mapping relationships among assets, investors can visualize their portfolios better and understand the risk associated with certain investments. This is especially helpful in identifying overlapping investments that may expose them to additional risks, or pinpointing synergistic opportunities that could maximize returns.
"Understanding the interconnectedness of investments is key to strategic decision-making, providing clarity where intuition may falter."
Additionally, network diagrams can be utilized for analyzing market trends, tracking the flow of funds, or assessing the impact of organizational changes. For instance, when a significant merger occurs, observing connections before and after the event can reveal shifts in power dynamics, market influence, or potential redundancies.
In summary, network diagrams are essential for visualizing and understanding complex relationships in both social and investment networks. They empower investors and analysts alike, making the invisible connections between entities clear, thereby facilitating informed decision-making.
Gantt Charts: Project Management Visualization
Gantt charts stand as a cornerstone in the realm of project management, providing a clear visual representation of project timelines, tasks, and their interdependencies. In the modern landscape, where effective time management is essential, understanding how to navigate and leverage Gantt charts enhances not just productivity but also strategic planning. Utilizing these charts shifts the focus to a structured approach, making it easier to monitor deadlines, resources, and responsibilities. The clarity that a Gantt chart offers is simply invaluable for teams working towards a common goal.
Gantt Chart Functionality
At its essence, a Gantt chart is more than just a bar graph plotted against a time scale. It lays out all project tasks along with their durations, start and end dates, and relationships to one another. This type of visualization aids project managers by allowing them to see which tasks can run concurrently and where bottlenecks may arise.
Key functionalities include:
- Task breakdown: Each task shows not only its duration but also its dependencies on preceding ones. This insight allows team members to plan their work effectively.
- Resource allocation: Project managers can easily distribute workloads across the team, avoiding situations where resources are overloaded.
- Visual tracking: Progress can be tracked visually, making it easy to see how close a project is to meeting its milestones.
By leveraging these aspects, Gantt charts empower teams to adjust their strategies in real-time, mitigating risks and enhancing performance.
Integration in Financial Project Planning
When it comes to financial project planning, Gantt charts play a pivotal role. They provide a systematic method to align project timelines with budget constraints, ensuring every dollar is spent wisely. Here are several ways Gantt charts integrate seamlessly into this field:
- Budgeting and scheduling alignment: By laying out tasks alongside financial milestones, organizations can gauge if the project is on track financially.
- Cash flow management: Gantt charts can help predict cash needs throughout the project by highlighting when certain expenses will occur, aligning them against incoming revenue.
- Stakeholder communication: A well-constructed Gantt chart serves as an excellent communication tool, helping stakeholders grasp project timelines and financial expectations quickly.
Incorporating Gantt charts into financial project planning is not just about visualizing tasks; it's about ensuring that every aspect of the project is orchestrated with meticulous precision, allowing for clear insights and enhanced decision-making.
"A Gantt chart isn't just a project tool; it's the compass guiding your financial planning toward success."
By understanding how Gantt charts function and the advantages they bring to financial project planning, both novice and seasoned investors can make informed strategic decisions, ultimately driving better outcomes.
Choosing the Right Graph Type
In the realm of data visualization, selecting the correct graph type is more than just a matter of aesthetics; it fundamentally affects how the audience perceives and interprets information. A well-chosen graph can transform raw data into a narrative that informs decisions, whereas a poorly selected one may obscure insights and provoke confusion. Whether we're analyzing stock trends or evaluating market demand, understanding how to choose the right graph type necessitates a thorough consideration of the underlying data and the audience's expectations.
This article explores the pertinent elements that contribute to making informed graphing decisions. Specifically, we'll delve into evaluating data characteristics and identifying audience needs. Both criteria are essential for determining which graph is best suited to communicate specific insights effectively.
Evaluating Data Characteristics
When grappling with data, the first step involves dissecting its characteristics. To choose the right type of graph, one must understand the fundamental properties of the data. Here are some factors to keep in mind:
- Data Type: Is it qualitative or quantitative? Categorical data lends itself well to bar charts, while numerical data is best visualized via line graphs or scatter plots.
- Distribution: What distribution does the data follow? Understanding whether the data is normally distributed, skewed, or categorical is pivotal in selecting the proper graph type.
- Trends and Changes: If the goal is to track a certain trend over time, line graphs or area graphs are great options. In contrast, if the focus is on establishing relationships among variables, scatter plots will usually work better.
Assessing these characteristics lays the groundwork for effective data representation. It ensures that the chosen graph not only displays information but does so in a way that enhances comprehension and insight. Information revealing trends or correlations is often lost without the proper context and format. Thus, evaluating data characteristics is not just prudent; it's essential for delivering clear and actionable insights.
Identifying Audience Needs
The second crucial consideration in graph selection revolves around understanding the audience. Tailoring a graph to meet the needs of its viewers can make or break a presentation or report. Here are some key questions to reflect upon:
- Audience Familiarity: What is the skill level of the audience? Are they financial experts or casual observers? Financial professionals might appreciate a detailed scatter plot to reveal correlations, while less experienced viewers might benefit from a simpler pie chart for insights on market share.
- Purpose of Visualization: What message needs to be conveyed? Understanding the ultimate goal will guide you in selecting a graph that resonates with and engages the audience. Do you want to demonstrate a trend, compare categories, or present a distribution? Each purpose aligns with different graph types.
- Aesthetic Appeal: How important is the visual appeal to the audience? Typically, simpler graphs with less clutter sway non-experts, while complex graphs may find a warm reception among seasoned professionals. However, elegance and clarity should always be the focus.
Ultimately, acknowledging audience needs is pivotal in shaping your graphical narrative. It's not just about depicting data; it's about fostering a connection between the data and the viewers. Adapting the visualization to the audienceβs level of understanding can significantly enhance message retention and impact.
"An informed choice regarding graph types can elevate a simple presentation into a compelling narrative."
The interplay between data characteristics and audience needs creates a robust framework for effective data visualization. Consider this approach next time you venture into the world of graphs, ensuring that every visual you create tells a story that is instantly graspable and impactful.
Emerging Trends in Graphing Techniques
In the ever-evolving landscape of data visualization, staying abreast of emerging trends in graphing techniques is imperative for both novice and seasoned investors. These trends not only reflect advancements in technology but also highlight shifting expectations in how data is presented and interpreted. As information becomes more complex and the need for instant comprehension intensifies, new graphing methodologies rise to the occasion, offering enhanced interactivity, aesthetic appeal, and analytical capacity.
Advances in Interactive Graphing
Interactive graphing has taken center stage, transforming the way users engage with visual data. Unlike static images, interactive graphs enable users to drill down into the data for a richer exploration of the underlying patterns and relationships.
One such advance is the integration of tools that allow users to manipulate data in real-time. This can be especially beneficial in finance, where conditions change rapidly. Imagine a stock market dashboard where investors can adjust time frames, modify variables, or zoom in on specific datasets. This level of interactivity not only enhances user experience but also leads to informed decision-making by allowing users to visualize what-if scenarios effectively.
Graph types like interactive bar charts and line graphs are staples in this domain. For example:
- Interactive Line Graphs: These can display historical data over various time periods, where the user can drag a slider to adjust the timeline, allowing a focused review of specific intervals.
- Interactive Scatter Plots: These visualizations may let users filter data points by relevant dimensions, such as sector or size, thus aiding in pinpointing correlations.
"Interactive graphs convert passive observation into active analysis, giving power back to the user."
Impact of Artificial Intelligence on Graphing
Artificial Intelligence (AI) has heralded a new era of data analysis and visualization. As graphic formats become increasingly complex, leveraging AI tools has become necessary. AI enhances graphing techniques by automating data analysis and ensuring accuracy, thus saving time and reducing human error.
Machine learning algorithms can analyze large datasets and uncover trends that might not be readily apparent. For instance, in the financial sector, AI can sift through historical financial performance to forecast future trends, visually represented through advanced graphing techniques. Some noteworthy applications include:
- Predictive Analytics: AI can create dynamic graphs that change in response to new data inputs, providing real-time analysis that is crucial for investments.
- Anomaly Detection: Graphing techniques powered by AI can quickly identify outliers or discrepancies in data, alerting investors to potential risks or opportunities previously missed.
In summary, as technology continues to evolve, the landscape of graphing techniques will reflect these changes. Interactive graphing enhances user engagement and comprehension, while AI integration promotes efficiency and deeper insights into complex datasets. Each of these trends invites a new level of sophistication in data analysis that is reshaping the financial decision-making landscape.