Essential Techniques in Data Analysis for Quantitative Research
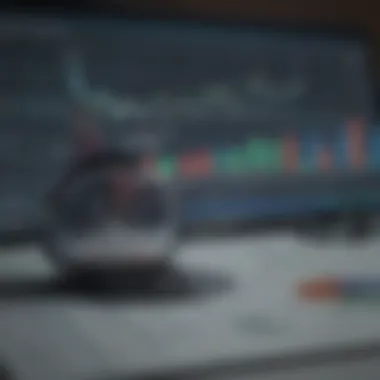
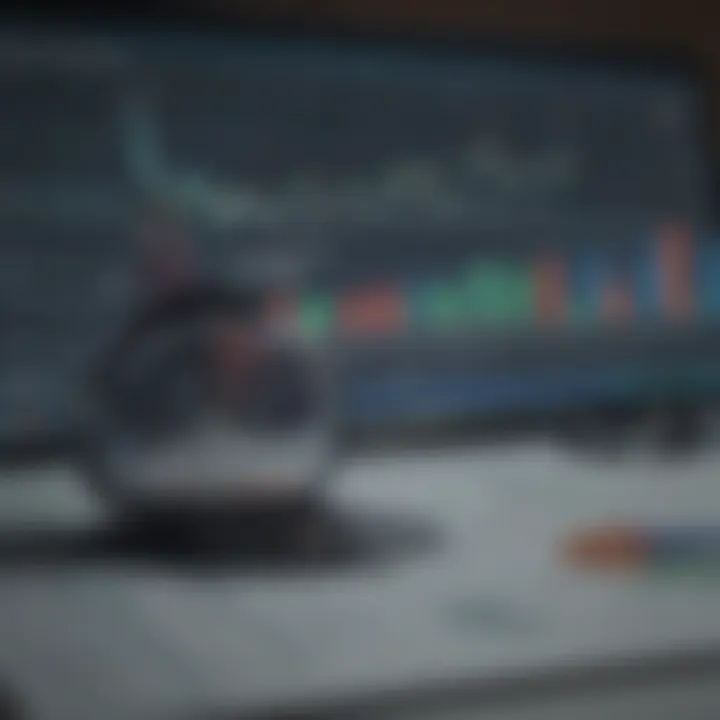
Intro
Data analysis stands at the heart of quantitative research, key for turning raw data into comprehensible insights. Itโs not merely about collecting numbers; itโs about understanding what those numbers signify. Researchers, both novice and experienced, often find themselves navigating a sea of statistics. The methodologies mentioned in this piece can be likened to a compass, guiding individuals toward meaningful conclusions from their data.
Investment Terminology
Understanding the language of data analysis is essential. Letโs break down some terms you might encounter along the way.
Key Terms and Definitions
- Data Set: A collection of related data points, essential for analysis.
- Quantitative Research: A systematic investigation that primarily focuses on quantifying relationships, behaviors, or phenomena through statistical software.
- Statistical Significance: A mathematical indication that the outcome observed in a study did not happen by chance.
- Hypothesis Testing: The process of making an assumption about a population parameter based on sample data.
Common Acronyms in Research
- ANOVA: Analysis of Variance, a method used to compare means across different groups.
- SPSS: Statistical Package for the Social Sciences, a software widely used for data management and statistical analysis.
- CI: Confidence Interval, a range of values thatโs likely to contain the true population parameter.
Expert Insights
This section will shed some light on practical strategies and essential tips that can enrich your analytical endeavors.
Data Analysis Techniques Overview
An understanding of various analytical methods is crucial. Here are some key techniques:
- Descriptive Statistics: Providing descriptive details about the data collected; it includes measures like mean, median, and mode.
- Inferential Statistics: Using a random sample of data taken from a population to make inferences about the population.
- Regression Analysis: A technique for modeling the relationship between a dependent variable and one or more independent variables.
"Statistics are like bikinis. What they reveal is suggestive, but what they conceal is vital." โ Aaron Levenstein
Tips from Data Analysts
- Always Clean Your Data: Without clean data, your analysis can yield misleading results. Take time to ensure your dataset is accurate and free of errors.
- Embrace Visualization: Sometimes numbers can be overwhelming. Tools like charts and graphs increase comprehension and aid in interpreting data effectively.
- Stay Updated on Software: The world of data analysis tools is dynamic. Keeping abreast of the latest software advancements can significantly enhance your analytical capabilities.
Preface to Quantitative Research
Understanding quantitative research is key to unlocking data-driven insights in numerous fields, from social sciences to market research. This form of research relies on numerical data, seeking to quantify variables, relationships, and phenomena. The emphasis is on measurement and statistical analysis, allowing researchers to draw conclusions that are both reliable and generalizable. With the increasing importance of data in decision-making, grasping the fundamentals of quantitative research becomes not just helpful, but essential.
Quantitative research often employs structured tools such as surveys or experiments, which collect data in a systematic and standardized manner. This structured approach helps in eliminating subjectivity, ensuring that the results can speak for themselves. This objectivity is a significant advantage over qualitative methods, which can sometimes cloud the purity of data interpretation.
Another profound element tied to this style of research is the ability to conduct comparative analyses. For instance, researchers can compare outcomes across different segments or demographics, explore correlations, and delve into causal relationships through rigorous methods. For those with a keen interest in exploiting statistical insights, understanding these comparisons enriches the research landscape.
"The beauty of quantitative research lies in its ability to take a complex world and translate it into numbers that tell a story."
Moreover, the implications of quantitative research stretch beyond academia; they resonate in business strategy, public policy, and healthcare analytics. Companies leverage such research to hone in on market trends, evaluate customer satisfaction, and fine-tune product offerings based on measurable feedback. This adaptability highlights its universal relevance.
However, diving into quantitative research brings along its considerations. The design of research must be thoughtful to avoid biases, ensure validity, and maintain reliability. Small missteps can skew results, so a solid grasp of the data collection process becomes crucial.
In the bigger picture, the introduction to quantitative research sets the stage for understanding why data analysis specifically is of utmost importance for accurate interpretation of that data. It paves the way for deeper involvement with analytical tools, statistical techniques, and ultimately, the informed conclusions that influence real-world decision making.
Key Concepts in Data Analysis
Data analysis comprises a myriad of essential concepts pivotal for conducting effective quantitative research. Understanding these concepts is not only vital for obtaining accurate results, but it also aids researchers in making informed decisions. Grasping the intricacies of population and samples, variables and measurement, and data types can make or break the research process. Each of these elements contributes significantly to the robustness of the analysis.
Population and Sample
The foundation of any quantitative study often lies in the distinction between population and sample. The population refers to the entire group that a researcher is interested in studying. For example, if youโre investigating the spending habits of teenagers in the US, your population includes all teenagers across the country. Conducting research on the entire population can be daunting and impractical due to factors like cost and accessibility.
This is where a sample comes into playโa segment of the population selected for analysis. A well-chosen sample can even represent the population's characteristics effectively. The act of sampling isn't just about grabbing a handful of individuals, though. It must be purposeful and systematic, often using techniques like random sampling or stratified sampling.
"A well-defined sample is a stepping stone to credible research results."
Understanding how to select a representative sample is crucial. If the sample is biased, it can skew results and conclusions, leading to misleading recommendations. Therefore, ensuring that the sample selection reflects the population's diversity is imperative.

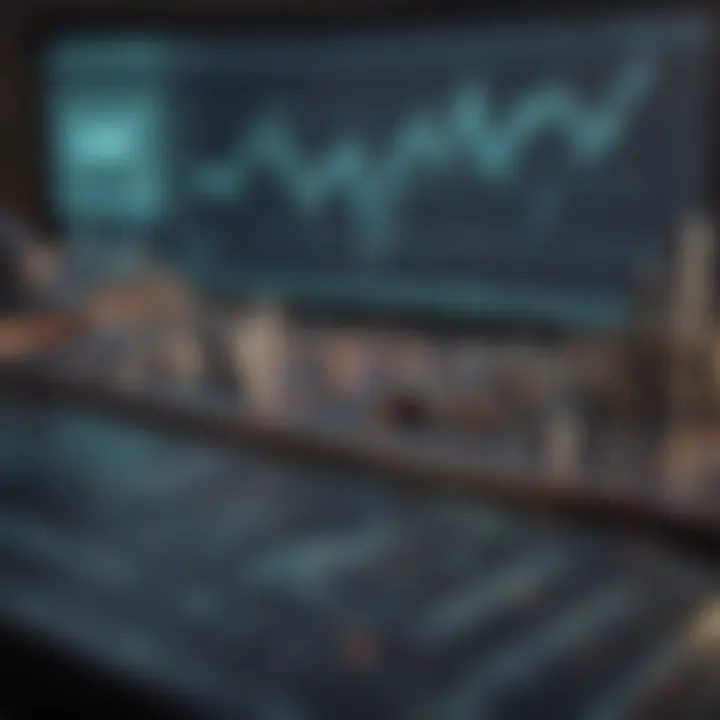
Variables and Measurement
In quantitative research, variables are the bread and butterโthey are the characteristics or properties that can take different values. For instance, in the earlier example regarding teenagers, variables could include age, income, or spending habits. There are two main types of variables: independent and dependent. The independent variables are those that researchers manipulate or measure to observe their effect on other variables. In contrast, dependent variables are what researchers aim to explain or predict.
Measurement of these variables is an equally vital process. It involves determining how these variables will be quantified. Researchers commonly use several scales of measurement, including nominal, ordinal, interval, and ratio scales. Each one has specific uses and implications regarding the types of statistical analysis that can be performed:
- Nominal: Categories with no inherent order (e.g., types of cuisine).
- Ordinal: Categories with a set order but unknown intervals (e.g., ranking in a competition).
- Interval: Numeric scales where intervals are meaningful, without an absolute zero point (e.g., temperature in Celsius).
- Ratio: Numeric scales that have an absolute zero, allowing for a full range of statistical operation (e.g., weight, height).
Choosing the appropriate measurement scale is crucial, as it impacts the statistical techniques that can be applied later.
Data Types and Their Implications
Understanding data types is another cornerstone of quantitative analysis. In general, data can be classified into two main types: qualitative and quantitative. While qualitative data captures non-numerical information such as opinions or experiences, quantitative data encompasses numerical values that allow for mathematical manipulation.
Quantitative data is further classified into:
- Discrete data, which comprises countable items (like the number of students in a class).
- Continuous data, which can take any value within a range (like the height of individuals).
The implications of these distinctions are significant. Different statistical tests, analyses, and interpretations apply based on whether data is discrete or continuous. Using the wrong type can lead to erroneous conclusions. Thus, it is important for researchers to identify these data types correctly before diving deep into analysis.
By familiarizing with these key concepts, researchers can lay a strong groundwork for their quantitative analyses. This understanding opens further discussions about tools and methodologies essential for effective data analysis.
The Data Collection Process
Data collection is the backbone of quantitative research, playing a critical role in shaping the results and conclusions drawn from a study. Without a systematic approach to gathering data, the findings can be misleading or, worse, entirely meaningless. Every researcher knows that the strength of their research lies not only in analyzing data but in the quality and relevancy of the data itself. Thatโs why understanding the data collection process mustn't be brushed aside.
The process involves careful planning, execution, and assessment to ensure that the data collected reflects the reality it aims to represent. There are several elements and considerations that serve as guiding principles in designing an effective data collection strategy, which are essential for obtaining reliable results.
Designing a Data Collection Strategy
Creating an effective data collection strategy is like laying the groundwork before building a house. If you donโt set a solid foundation, everything built upon it can easily collapse.
- Define your objectives: Before anything else, identify what you want to achieve. Are you exploring a hypothesis, or simply describing trends? This will shape your entire strategy.
- Choose your method: Will you use surveys, interviews, observations, or experiments? Each method has its pros and cons, influenced by your objectives and available resources.
- Set your timeline: Establishing a timeline for data collection helps clearly organize the process and avoid inevitable delays.
- Select your sample: Determine who will be part of your study. This shouldn't be an afterthought. The variety and size of your sample can dramatically affect the outcomes of your research.
A well-thought-out strategy ensures that the data you collect aligns closely with your research goals, leading to relevant results.
Survey Methods and Instruments
Surveys are among the most popular methods for collecting quantitative data, providing a structured approach to gather feedback, opinions, and insights from a study population. However, it shouldn't be taken lightlyโdesigning a survey is both an art and a science.
- Question types: Decide on the types of questionsโclosed or open-ended. Closed questions are easier to quantify, while open-ended questions may yield more nuanced insights but are tougher to analyze.
- Scale and format: Consider using Likert scales or multiple-choice formats, making it simple for respondents to express their opinions or experiences quickly.
- Distribution platforms: Utilize online tools like Google Forms or SurveyMonkey for ease of data collection and analysis. These platforms facilitate administration and aggregate data seamlessly.
Remember, a well-crafted survey yields high-quality data, which can stand the test of statistical analysis.
Data Quality and Reliability
The integrity of your data hinges on quality and reliability. Poor-quality data can compromise your research entirely. To ensure that your study is built on solid ground, focus on the following:
- Pilot testing: Conduct preliminary tests of your instruments with a smaller sample. This can help you identify potential issues and refine your approach.
- Consistency checks: Continuously monitor data collection to identify and rectify errors promptly. Even small mistakes can lead to larger discrepancies in the findings.
- Training of data collectors: If your research involves multiple personnel, ensure that everyone is on the same page regarding how to administer the survey or collect data.
As a takeaway, high-quality data does not just happen by chance; itโs the result of thoughtful planning and execution. With each step in the data collection process properly considered, your quantitative research can lead to valid and generalizable conclusions, serving its purpose effectively.
"Good data collection is critical to ensuring your findings arenโt just a lucky guess, itโs the difference between insightful research and mere conjecture."
As you further your exploration into the realm of quantitative research, remember that each selection made during the data collection process carries significant weight and consequences on your overall results.
Analytical Tools and Software
The use of analytical tools and software is paramount in the realm of quantitative research. They significantly enhance the efficiency, accuracy, and sophisticated depth of data analysis, lending researchers the ability to distill raw data into meaningful insights. Without these tools, attempting to navigate the vast ocean of numbers and trends can be akin to finding a needle in a haystack. The right software acts not just as a calculator but as a trusted advisor in the journey from data collection to hypothesis testing.
One of the distinguishing benefits of employing analytical tools is their capability to handle large datasets that might overwhelm traditional analysis methods. These tools can automate complex calculations and visualizations, freeing researchers to focus on interpreting results rather than becoming bogged down in numbers. Furthermore, many tools are designed to minimize the likelihood of human error, ensuring that conclusions drawn are not merely a reflection of miscalculation.
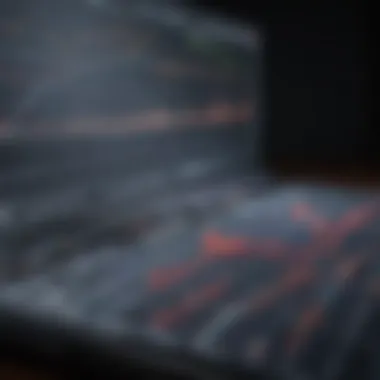
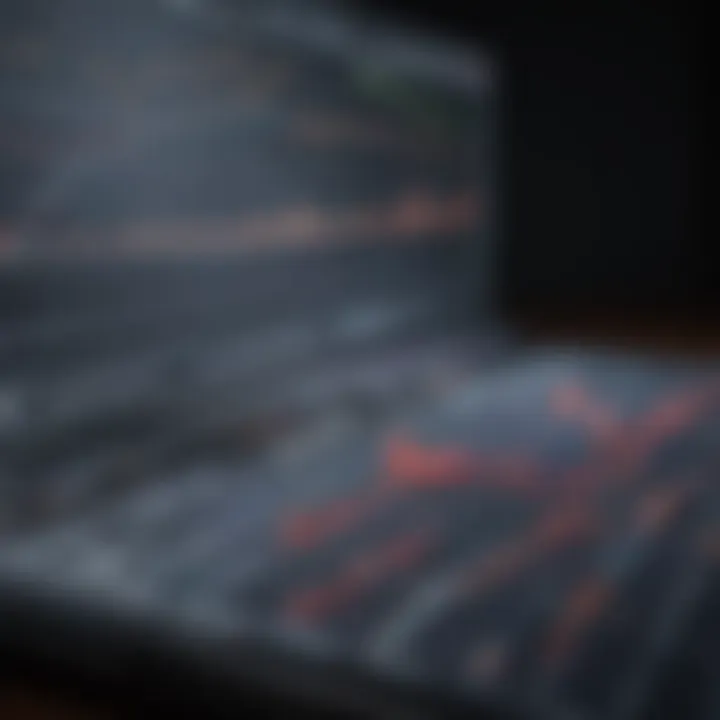
Statistical Software Options
Statistical software options are characterized by features that cater to varying complexities of data analysis. SPSS and SAS stand out in the world of statistical software, offering robust solutions for handling diverse statistical analyses. They allow users to perform extensive functions, from basic descriptive statistics to more complex multivariate analyses.
Not only do these tools allow for advanced statistical tests, but they also tend to come with user-friendly interfaces. This synergizes well with training various levels of research staff, thus equipping them with skills that might otherwise take considerable time to develop. The ease of data importation and management within these platforms is another boon, allowing researchers to concentrate on data integrity instead of mere data entry. Additionally, support forums for these platforms provide invaluable help, often making troubleshooting a manageable task.
Spreadsheet Tools
While they may not possess the mighty capabilities of specialized statistical software, Excel and Google Sheets are indispensable allies for data analysis. These tools serve as a fundamental entry point for many researchers. They can efficiently handle numerical data and are often supplemented by statistical add-ons to elevate their functionality.
When it comes to basic data manipulation, spreadsheets allow for quick calculations, chart generation, and even pivot tables, which help in segmenting data effectively. However, users must be cautious; for larger datasets, performance can become an issue. Thus, while well-suited for basic analyses and small surveys, larger-scale research may require the integration of more cutting-edge software solutions.
Programming Languages for Data Analysis
Venturing into programming languages like R and Python offers researchers a flexible approach to data analysis. These languages excel in managing large datasets and conducting intricate operations that might elude more traditional methods. Researchers equipped with programming knowledge can manipulate data in ways that pre-packaged software may not allow.
The climate of data science has made R and Python particularly prevalent, with a plethora of libraries available for everything from data manipulation to machine learning. For instance, R's and Python's provide robust options for visualizing trends and patterns within datasets.
Engagement in programming for data analysis can appear daunting to newcomers, but the online community is robust. Numerous resources and forums exist where enthusiasts from various skill levels share insights, resolve queries, and encourage collaborative learning.
Statistical Analysis Techniques
Statistical analysis techniques are the backbone of data interpretation in quantitative research. They provide a structured approach to extracting meaningful insights from vast datasets, enabling researchers to not only comprehend their findings but also to convey them effectively to their audiences. Different techniques serve unique purposes, whether it's summarizing data through descriptive statistics, making inferences about populations based on samples, or exploring relationships between variables using regression analysis. Understanding these techniques is crucial for anyone involved in quantitative research, ensuring that data-driven conclusions are robust and insightful.
Descriptive Statistics
Descriptive statistics are fundamentally about summarizing and organizing data so that it is easily understandable. At their core, they assist researchers in grasping the general characteristics of data sets. For instance, imagine you're analyzing student test scores from a sizable school district. Descriptive statistics would allow you to calculate averages, determine the range of scores, and even identify the modeโthe score that appears most frequently.
Key Elements of Descriptive Statistics:
- Measures of Central Tendency: These include the mean, median, and mode. Each measure gives a different perspective on the dataset's center.
- Measures of Dispersion: This concept dives into how spread out the data is, looking at ranges, variance, and standard deviations.
- Visual Representation: Tools like histograms and box plots help visualize data distributions, making it easier for stakeholders to grasp key findings at a glance.
"Descriptive statistics turn a mountain of numbers into a hill you can walk over. They highlight whatโs important, hidden in plain sight."
Overall, descriptive statistics lay the groundwork needed for more complex analyses, offering a clear snapshot of the data's landscape.
Inferential Statistics
Moving beyond mere description, inferential statistics allow researchers to make predictions or generalizations about a population based on a sample. This leap is vitalโespecially when itโs impractical to study an entire population.
Consider this: if a health researcher surveyed a sample of 500 individuals about their dietary habits, inferential statistics could help project those findings to a broader population of 50 million. By employing techniques like confidence intervals or hypothesis testing, one can assess how likely it is that the sample's characteristics genuinely reflect the larger group.
Key Points to Consider in Inferential Statistics:
- Sampling: The selection of a representative sample is crucial. Biases can lead to skewed interpretations.
- Confidence Levels: A typical confidence level is 95%, meaning researchers can be 95% certain that their results are not due to chance.
- P-values: These indicate the probability that the observed data would occur if the null hypothesis were true. A smaller p-value suggests stronger evidence against the null.
Inferential statistics offer the penultimate tools to navigate uncertainty in research, allowing analysts to project their findings and thus influence decisions based on sound evidence.
Regression Analysis
Regression analysis goes to the heart of understanding relationships between variables. At its essence, it helps researchers figure out how one variable affects another. For instance, in studying the impact of study hours on student performance, a regression analysis can reveal not just if there's a correlation, but the extent and nature of that relationship.
Various Aspects to Consider in Regression Analysis:
- Linear vs. Non-linear: Depending on the relationship, analysts can choose a linear model for straightforward associations or more intricate models for complex data.
- Coefficient Interpretation: Each coefficient in a regression equation indicates the expected change in the dependent variable for a one-unit change in the predictor variable.
- Multicollinearity: It's vital to check if predictor variables are correlated with each other, as this can distort the analysis.
In summary, regression analysis envelops researchers in understanding intricate relationships, paving a path to more precise and actionable insights.
Interpreting Data Results
Understanding how to interpret data results is a cornerstone of quantitative research. This section unpacks the significance of data interpretations, discussing their utilization and practical implications in research contexts. The ability to derive insights from raw numbers can shape conclusions and inform decision-making, making it essential for researchers at all levels.
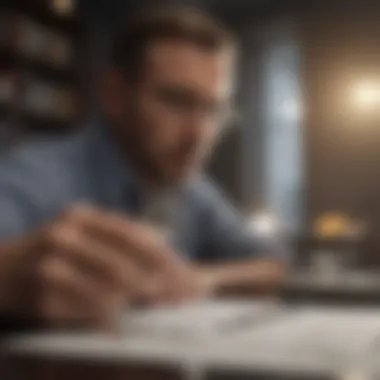
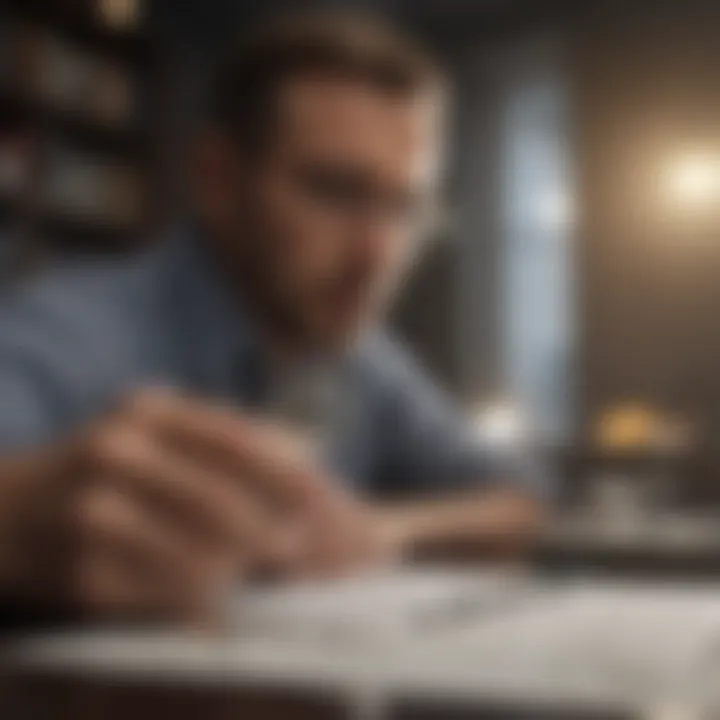
Understanding Statistical Significance
Statistical significance helps determine whether the results of an analysis are likely due to chance or if they have real-world implications. In simpler terms, it tells researchers if their findings are reliable and worth trusting. Commonly, a p-value is used to express this concept, with values less than 0.05 typically indicating significance.
However, this isn't just about getting the right number. It's crucial to appreciate what that number represents. For instance, a p-value of 0.03 signifies a 3% chance that the findings happened randomly, suggesting a strong likelihood that the research results reflect the true state of affairs, particularly in studies concerning medicine or social behavior.
But never put all eggs in one basket solely based on statistical significance. It's a guiding star, not the only consideration. Researchers must also think about the context of their work and the practical relevance of their results.
Effect Size and Practical Significance
While statistical significance focuses on whether a finding exists, effect size tells us how important that finding is. Effect size offers a measure of the strength or magnitude of a phenomenon. For instance, in a study analyzing the impact of a new teaching method, a statistical significance might emerge, but effect size will tell us if the method really changes student outcomes noticeably or just slightly.
Practical significance contrasts sharply with mere statistical significance. Consider the example of a new drug: if it lowers symptoms significantly, the effect size should reveal how much better patients feel compared to those on a placebo.
In practice, recognizing both statistical and practical significance aids informed decision-making.
The Use of Visualizations
Visualizations serve as powerful tools in data interpretation. They convert complex results into easily digestible formats, allowing researchers to convey their findings effectively. Everyone knows a picture tells a thousand words; this holds true in quantitative research too.
Graphs, charts, and tables can highlight trends, relationships, and patterns that words alone may struggle to express. For example, a scatter plot illustrates correlations beautifully, whereas a bar chart can succinctly compare group means at a glance.
Hereโs how visualizations enhance understanding:
- Clarify Findings: Helps remove ambiguity in results.
- Engage Viewers: A well-crafted visualization can captivate the audience, guiding them through complex data.
- Promote Retention: People tend to remember visual information better than textual data.
"A well-designed graph is worth a thousand tables."
In sum, data interpretation is an intricate dance of significance, size, and representation, weaving together a coherent narrative that ultimately informs action. By appreciating the details, researchers can ensure their work doesnโt just exist in isolation but contributes meaningfully to their fields.
Limitations of Quantitative Research
Understanding the limitations of quantitative research is crucial within the context of data analysis. While quantitative methods provide a structured approach to data collection and analysis, they come with their own set of challenges that researchers must recognize. By acknowledging these limitations, researchers can make informed decisions and enrich their studies with complementary insights where necessary. Here, weโll delve into prevalent biases and the often-overlooked qualitative insights that can complement quantitative findings.
Potential Biases
Bias in quantitative research can stealthily alter the outcomes of a study. Itโs akin to trying to see the forest through a foggy lens; without clarity, important details may be lost. Potential biases can arise from various sources, such as:
- Sample Bias: This occurs when the participants chosen for the study are not representative of the larger population. An example might be surveying college students to understand national voting patterns. The results would be skewed since they donโt represent all demographics.
- Measurement Bias: If the tools used to collect data are flawed, the outcomes will reflect those flaws. For instance, a poorly designed survey question can lead to misleading data. An ambiguous question can confuse respondents, producing inconsistent answers.
- Response Bias: Sometimes, individuals might respond to surveys or experiments in ways that align with perceived societal norms rather than their true beliefs. An instance is when respondents might overstate their exercise habits for fear of judgment.
Recognizing these biases can help in attempting to mitigate their effects. Itโs vital for researchers to rigorously evaluate their methods and consider conducting follow-up studies to cross-check findings.
Overlooking Qualitative Insights
While quantitative research emphasizes numbers and statistics, it can often ignore the rich tapestry of human experience captured in qualitative data. Qualitative insights can provide depth and context that numbers alone may fail to convey. Here are some key reasons why qualitative research should not be sidelined:
- Contextual Understanding: Numbers can provide trends, but stories convey the reasons behind those trends. For instance, tracking ice cream sales might show a spike during summer months. However, understanding customer preferences, celebration events, or regional flavors could paint a clearer picture of these patterns.
- Exploratory Analysis: Qualitative methods, like interviews or focus groups, allow researchers to explore new concepts. When faced with surprising data outcomes, qualitative insights can lead to further inquiries that enrich the original study.
- Participant Voices: Quantitative data can sometimes mask the individual voices of participants. Qualitative research provides a platform for these voices, allowing for a more holistic view of the subject matter.
In essence, while quantitative methods offer a robust framework for analysis, incorporating qualitative perspectives can yield richer, more nuanced interpretations. In the world of data analysis, flexibility and adaptability are essential to harnessing the true potential of research efforts.
By blending both quantitative and qualitative research, one can create a more complete narrative that goes beyond mere data points.
The End
Key Takeaways from Data Analysis
The process of analyzing data can be intricate but understanding the fundamental takeaways enhances clarity. Firstly, grasping the importance of proper data collection forms the bedrock of any robust analysis. Without reliable data, the findings may end up leading researchers astray. In addition, familiarizing oneself with various analytical tools helps elevate the quality of research outcomes by streamlining workflows and enhancing precision. Here's a closer look at crucial insights:
- Understand the significance of statistical methods: Recognizing various techniques like descriptive and inferential statistics can empower researchers to extract meaningful insights from raw data.
- Data visualization is key: Engaging with tools that visualize data aids in comprehending complex information, helping to convey findings more effectively to a broader audience.
- Practical implications of results: Reflecting on how data findings impact real-world scenarios drives research relevance and fosters informed decision-making.
"The past cannot be changed, but the future is in your hands."
The Future of Quantitative Research
Looking ahead, the landscape of quantitative research is poised for transformation, driven by technological advancements and evolving methodologies. The integration of AI and machine learning is set to redefine how researchers approach data analysis, making it more efficient and insightful. Additionally, embracing open data initiatives will enable broader accessibility, encouraging collaboration across various disciplines. Future trends could shape quantitative research in the following ways:
- Greater emphasis on interdisciplinary approaches: Seamless integration of techniques and knowledge from distinct fields enhances the robustness of research.
- Real-time data analysis: With the surge of data collection in real-time, researchers will adapt to analyze data on-the-go, transforming how conclusions are drawn.
- Ethical considerations: As the reliance on data grows, so will the focus on ethical practices related to data acquisition and usage, ensuring responsible research is prioritized.
In summary, as the research environment evolves, the role of data analysis continues to grow in significance. By staying abreast of new developments and adapting strategies accordingly, researchers can position themselves at the forefront of this dynamic field.